Social neuroscience for education
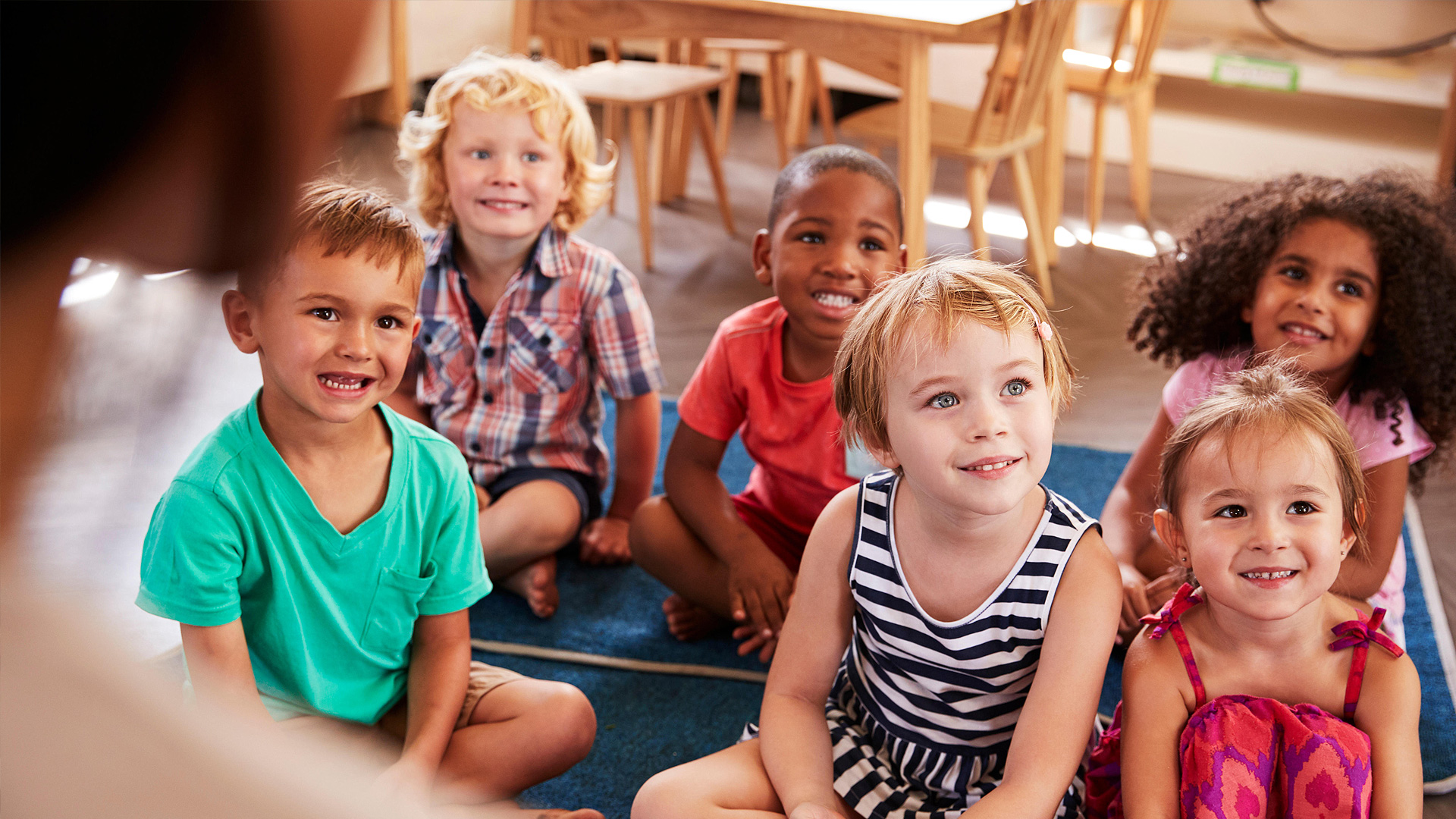
Executive summary
- The ‘social brain’ is a set of brain regions that supports the processing of social information as well as non-social functions.
- An example of a social ability necessary for interaction with other people is face recognition, which has been widely studied in neuroscience.
- Social skills and emotional intelligence, including face recognition, predict academic achievement.
- Social cognition also engages more complex processes such as learning from the actions of others, making decisions in groups, and theory of mind (inferring others’ intentions).
- Humans are motivated by concerns that go beyond payoff maximization: from altruism and fairness to envy, status and social norms.
- This can be studied using economic games in which multiple players interact simultaneously or sequentially.
- These social processes can be studied using experimental games from game theory combined with fMRI.
- Overall, research indicates that neuroeconomics tools can be used to study different aspects of social cognition, including complex social interactions.
Introduction: social neuroscience
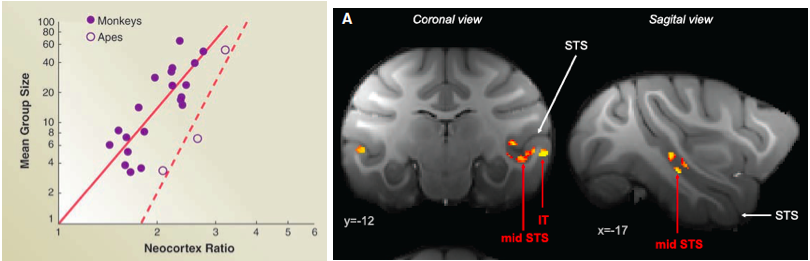
Figure 1. Left: illustration of the social brain hypothesis. From Dunbar & Shultz, 2007. Right: gray matter positively correlated with social network size in the temporal lobe and rostral prefrontal cortex (Sallet et al., 2011).
All primates live in complex social structures where the demands of cooperation and competition must constantly be balanced. These demands are likely to have influenced the evolution of the human brain. The “social brain hypothesis” proposes that the need to optimize behavior within complex social environments significantly influenced the evolution of primate brains. This theory is supported by evidence for the co-evolution of social complexity and brain structures (Dunbar, 1992) (see Figure 1, left). For example, the relative size of the neocortex has been reported to correlate with the size of an individual’s social network (Dunbar & Shultz, 2007). More recent studies have proposed that the size of the amygdala, a key brain region involved in processing emotion, is larger in people who have regular contact with a larger number of relatives, friends, and colleagues (Bickart et al., 2011). Although the human prefrontal cortex is larger than those of closely related primate species, it is less the relative size than the functional organization in terms of neural circuitry and connectivity with other brain structures that determines cognition (Semendeferi et al., 2002; Teffer & Semendeferi, 2012). Recent studies indicate that the human cognitive system could have evolved to be highly flexible to optimize interactions with other members of the group. One possible cause for the correlation between social network size and increases in brain structures comes from recent primate studies showing that variation in adult rhesus macaques’ social environments cause changes in brain structure in a distributed neural circuit centered on mid-superior temporal sulcus (STS), anterior cingulate cortex (ACC) and rostral prefrontal cortex (rPFC) (Sallet et al., 2011) (see Figure 1, right). These studies exploited the pseudo-randomized assignment of individual animals to social groups within a colony.
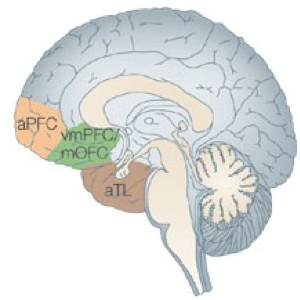
Figure 2. The social brain networks include brain regions activated by reward and valuation, frequently identified in studies of social interaction within the frame of reference of the subject’s own actions: anterior cingulate cortex sulcus (ACCs), ventromedial prefrontal cortex (VMPFC), amygdala, and ventral striatum (VStr). Other brain regions are activated by considering the intentions of another individual: anterior cingulate cortex gyrus (ACCg), dorsomedial prefrontal cortex (DMPFC), temporoparietal junction (TPJ), and superior temporal sulcus (STS).
The neural circuits involved in decision-making in a social setting have been identified (see Figure 2) (Behrens et al., 2009; Kane et al., 1999). Key components include the orbitofrontal cortex (OFC), the ventromedial prefrontal cortex (vmPFC), the dorsomedial (dmPFC), and the dorsolateral prefrontal cortex (DLPFC), parts of the superior temporal sulcus (STS), including a region near the temporo-parietal junction (TPJ), and the anterior cingulate gyrus (ACCg). Moreover, there is extensive evidence that social decision-making relies on many “non-social” subcortical and brainstem circuits, such as the medial prefrontal cortex (mPFC), the superior temporal sulcus (STS) and the temporo-parietal junction (TPJ) (see Figure 2). This does not mean that brain regions considered ‘social’ only process social information. In fact, recent studies have highlighted the need to distinguish between cognitive and neural processes that are specialized in social behavior. Processes can be socially specialized at the implementational, computational and algorithmic levels (Lockwood et al., 2020). To understand how specific social behavior compares to ‘non-social’ processes, we first need to understand the social goal (are we cooperating, learning from, or helping the other person or group?). Second, we need to understand the algorithm by which this is achieved (e.g., reinforcement learning, cost-benefit trade-off, etc.). Third, we need to know how the social process is implemented, and in which brain areas, circuit, or cell it is realized. A distinction is needed between social and non-social processes, either at the level of algorithm or of implementation, to conclude that there is social specificity. We have proposed that a similar distinction applies to understanding moral cognition (Qu et al., 2022; see also IBRO brief on moral decision-making by JC Dreher).
Social skills and emotional intelligence predict academic achievement
How can social neuroscience inform classroom teaching? How can we apply the findings of social neuroscience to optimize different forms of learning? Supportive, encouraging, secure and caring relationships between educators and students stimulate students’ neural circuitry, priming their brains for neuroplastic processes. Such relationships serve emotional regulation, which enhances learning. An environment that maximizes attention and motivation increases learning.
There is ample evidence that emotion knowledge and social skills are key abilities that young children must develop at the beginning of their schooling to ensure future academic success (Cavadini et al., 2021). ‘Emotion knowledge’ refers to the capacity to recognize emotions from faces and various behavioural cues and social contexts. Several studies have demonstrated that emotion knowledge is linked to social competence and academic achievement in young children (Ursache et al., 2020; Waiden & Field, 1990). For example, a recent meta-analysis of studies with children (aged 3 to 12) revealed that students with higher levels of emotion knowledge tend to have higher academic performance, as well as better peer acceptance and school adjustment (Voltmer & von Salisch, 2017). The recognition of facial expressions of emotion is key to the development of emotional understanding and adapted social interaction. For example, the ability to recognize and label expressions of emotion at age 5 is a long-term predictor of social behavior and academic performance (Izard et al., 2001). The quality of relationships with teachers and peers at the beginning of the preschool year also predicts increases in emotion knowledge and academic achievement at kindergarten (Torres et al., 2015). Together, these results suggest that emotion knowledge predicts socio-behavioral development and academic achievement in young children.
Other studies have revealed the influence of social competence on academic performance. For example, a longitudinal study of children aged between 5 and 8 showed that social understanding at age 5 predicted social competence at age 7, which in turn predicted school achievement at age 8. Social competence also mediated the association between early social understanding and later school achievement (Lecce et al., 2017).
Social processing and the recognition of emotion in faces
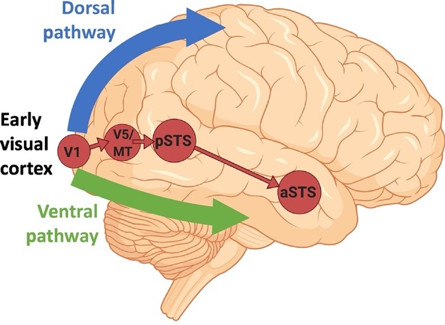
Figure 3. According to recent studies, the ventral pathway computes the identity of an object, the dorsal pathway computes the location of an object and the actions related to that object, and a third pathway is specialized in the dynamic aspects of social perception (Pitcher & Ungerleider, 2021) (expressions, eye-gaze, audio-visual integration, intention, and mood). This latter pathway emerges from the early visual cortex, via motion-selective areas, into the superior temporal sulcus (STS).
Because emotion recognition is linked to social competence and academic achievement in young children, it is vital to understand the visual pathways underlying visual and emotional processing. Early research identified a distinction between a ventral stream that processes ‘what’ is perceived and a dorsal stream processing either spatial perception (‘where’) or non-conscious visually guided action (‘how’) (Mishkin et al., 1983) (see Figure 3). According to this view, the ventral pathway computes the identity of an object while the dorsal pathway computes its location and the actions related to that object. More recently, it has been proposed that two pathways emerge from the dorsal stream. These consist of projections to the prefrontal and premotor cortices, and a projection to the medial temporal lobe that passes both directly and indirectly through the posterior cingulate and retrosplenial cortices (Kravitz et al., 2011). These pathways support visuospatial processing relative to spatial working memory, visually guided action and navigation. A recent study proposed that a third pathway is specialized in the dynamic aspects of social perception (Pitcher & Ungerleider, 2021) (expressions, eye-gaze, audio-visual integration, intention, and mood). This pathway projects from the early visual cortex, via motion-selective areas, into the superior temporal sulcus (STS).
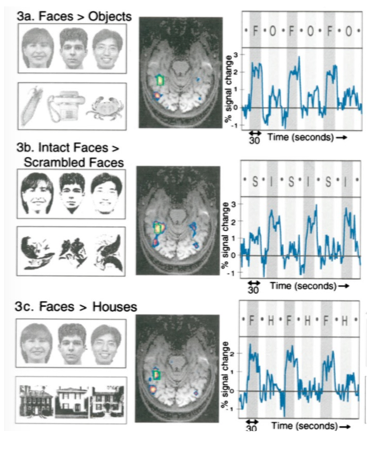
Figure 4. Classical finding obtained in humans using fMRI when viewing different categories of objects and faces. The fusiform area: a module in human extrastriate cortex specialized for face perception (Kanwisher et al., 1997).
A number of fMRI studies in humans have revealed that there is a region of the brain selectively engaged by faces. Located in the ventral temporal cortex, it is known as the fusiform face area (FFA), and it responds more strongly to faces compared to objects (see Figure 4). Subsequent fMRI studies performed in macaques showed face-selective regions when comparing images of faces versus non-face objects. Faces selectively engaged several regions in the macaques’ IT cortex, known as face patches (see Figure 5). This led to direct electrophysiological recording from single cells in fMRI-identified face patches, which allowed researchers to identify the selectivity of cells in these patches. fMRI experiments have revealed at least six face-selective regions in each hemisphere: the posterior lateral (PL), middle lateral (ML), middle fundus (MF), anterior lateral (AL), anterior fundus (AF) and anterior medial (AM) patches (Hesse & Tsao, 2020). Most neurons that are visually responsive from these face patches are strongly face-selective. These findings indicate that there is a distributed face-processing network in the temporal cortex, the frontal cortex and the amygdala coding faces. Within the IT cortex, while neurons responsive to faces are found throughout ventral temporal cortex, they are concentrated within fMRI-identified face-selective regions. These fMRI-identified face regions reflect both greater proportions of face-selective neurons and greater selectivity of those neurons.
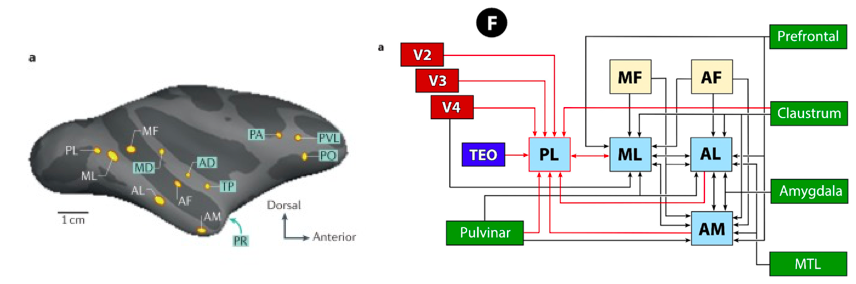
Figure 5. Left: face patches show increasing invariance to view changes going from middle lateral (ML)/middle fundus (MF) to anterior lateral (AL) to anterior medial (AM) face patches. Right: connectivity pattern between early visual areas and face patches.
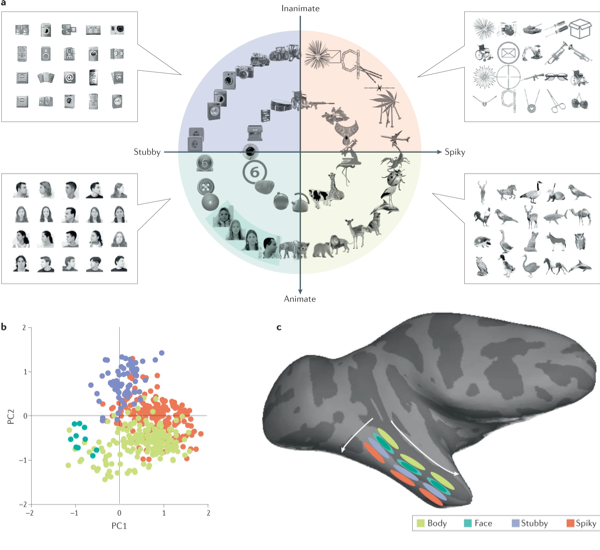
Figure 6. (a) Recent findings in monkeys show four quadrants after separation by two principal components (PCs) of object space, animate-inanimate components (PC1) and stubby-spiky components (PC2). The stimuli in each of the quadrants were used to map four networks (face patches, body patches, spiky patches and stubby patches) using functional MRI. (b) Projection of preferred axis of each cell onto PC1 versus PC2 for all neurons recorded across four networks (spiky network: orange, body network: lime, face network: green, stubby network: blue). (c) Schema showing the threefold-repeated topographic map in the inferotemporal cortex that is organized according to the four quadrants of object space.
The neural coding of identity may not be unique to faces. Recent research has used deep networks to understand computations in face patches, enabling precise testable model prediction of the representation of faces (Hesse & Tsao, 2020). A recent study investigated the overall large-scale organization of the IT cortex using a deep network (Bao et al., 2020), building a parametric object space by computing the first principal components of responses of units to a large set of objects. IT cells were clustered to form a coarse topographic map of the first two dimensions of this space. This map consisted of four quadrants encompassing face patches, body patches and two newly discovered networks; one representing ‘spiky’ objects and another ‘stubby’ objects (see Figure 6). Patches within the two newly discovered networks are anatomically connected as face and body networks. Cells in each network approximately projected incoming objects, formatted as vectors in object space, onto specific preferred axes, with the sign of the first two components of this axis attributed by the cell’s anatomical position in the IT cortex.
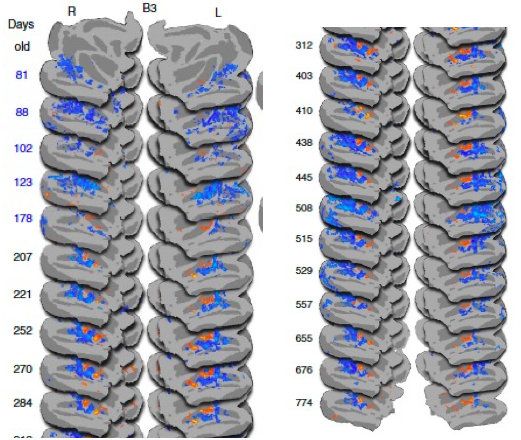
Figure 7. Emergence of face patches during brain development (Livingstone et al., 2017).
Face recognition emerges in infancy, yet the development of the neural mechanisms supporting this behaviour is largely unknown. A recent fMRI study in macaque monkeys measured brain responsiveness to faces, scrambled faces, and objects in the inferotemporal cortex (IT) between the ages of 1 month and 2 years. During this period, selective responsiveness to faces emerges. Interestingly, some functional organization is present at 1 month and face-selective patches emerge over the first year of development and are remarkably stable. Face selectivity is refined by a decreasing responsiveness to non-face stimuli (Livingstone et al., 2017) (see Figure 7).
Learning from others: neural systems engaged in social learning
Although emotion and face recognition may be considered as building blocks for efficient social interactions, social cognition is not restricted to these two aspects. In recent years, researchers have used tools developed in machine learning to investigate how humans learn from others, how they make decisions during social interactions, and how they make strategic inferences based on theory of mind (the capacity to attribute intentions to others).
Neural basis of learning from others
Recent research indicates that learning from others (actions and/or outcomes) engages specific brain systems compared to learning by oneself (i.e., receiving direct feedback for one’s actions or outcomes) (Joiner et al., 2017). There are also common neural mechanisms for social and non- social learning. Social learning follows the same principles as self-experienced value-based learning, including computations of the so-called prediction error signal (encoding the discrepancy between predicted and effective outcome). One early form of observational learning based either on observing others’ actions (Olsson et al., 2020) or their outcomes is imitation. Imitative learning typically involves a child copying the behaviors of an adult. Social learning occurs when the learner observes another agent act or receive specific outcomes (e.g., vicarious rewards) (see Figure 8). In this way, even without any practice or direct reinforcement the learner learns to perform the observed behavior. Thus, the learner is able to acquire new knowledge or skills from watching others’ experienced outcomes, such as through vicarious reinforcement.
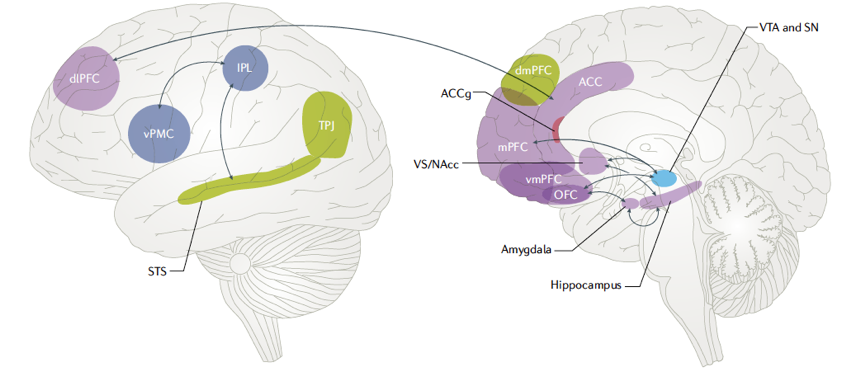
Figure 8. Social reward learning shares neural mechanisms with self-experienced learning. The light blue- shaded areas refer to regions involved in non-social reward learning while the red-shaded area refers to the ACC gyrus involved in vicarious reward learning. Regions involved in both social and non-social reward learning are represented by purple shading. Green-shaded areas refer to regions implicated in processing of social information, such as the understanding and attribution of mental states, while dark blue-shaded areas refer to core regions of the action/mirror network.
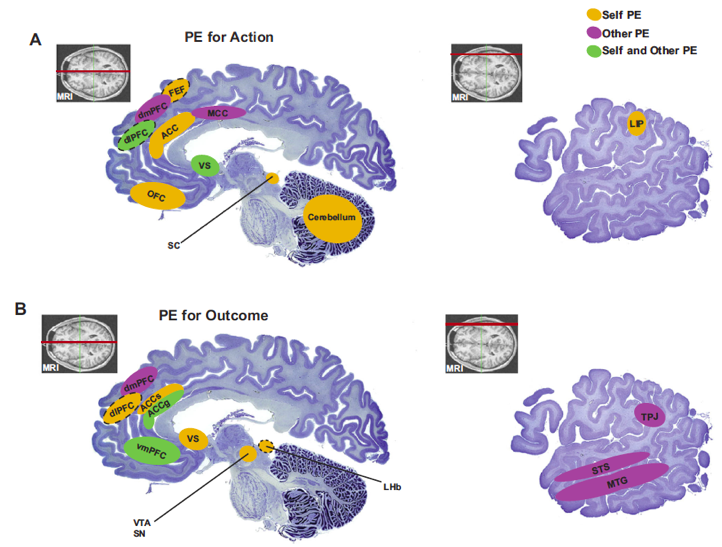
Figure 9. Key brain regions that have been shown to correlate with self-referenced prediction errors (in yellow) or other-referenced prediction errors (in purple), or both kinds of prediction errors (in green) in the domain of actions (A) and reward/value outcomes (B). ACC (anterior cingulate cortex), ACCg (anterior cingulate gyrus), ACCs (anterior cingulate sulcus), dlPFC (dorsolateral prefrontal córtex), dmPFC (dorsomedial prefrontal córtex), LHb (lateral habenula), LIP (lateral intraparietal area), MTG (medial temporal gyrus), OFC (orbitofrontal córtex), SC (superior colliculus), SN (substantia nigra), STS (superior temporal sulcus), TPJ (temporoparietal junction), vmPFC (ventromedial prefrontal córtex), VS (ventral striatum), VTA (ventral tegmental área) (Joiner et al., 2017)
Self-referenced and other-referenced reward prediction errors can be found across multiple brain structures that allow reinforcement learning algorithms to mediate social learning. Prediction-based computational principles in the brain may be conserved between self-referenced and other-referenced information (Joiner et al., 2017). One can thus distinguish between brain regions that correlate with self-referenced prediction errors, with other-referenced prediction errors, or with both kinds of prediction errors in the domain of actions (a) and reward/value outcomes (b) (see Figure 9).
Social learning is not a passive process of merely copying and trusting others. A recent review argues that both learning from others (social learning) and helping others learn (teaching) can be characterized as probabilistic inferences guided by an intuitive understanding of how people think, plan, and act (Gweon, 2021). Even young children draw rich inferences from evidence provided by others and generate informative evidence that helps others learn. By studying social learning and teaching through a common theoretical lens, inferential social learning provides an integrated account of how human cognition supports the acquisition and communication of abstract knowledge.
Social interactions: insights from game theory and neuroeconomics
Social interactions can be studied with economic game modeling situations in which future outcomes depend on individual choices (van Dijk & De Dreu, 2021). Economic games combined with fMRI and neurocomputational modelling allow researchers to identify the cerebral mechanisms underlying cooperation and coordination, including social preferences, norm enforcement, cooperative beliefs, signaling, reputation, and direct as well as indirect reciprocity. A classical example is the ‘ultimatum game’, an experimental economics game in which two parties interact anonymously and only once. The first player proposes how to divide a sum of money with the second party. If the second player rejects the proposed division, neither receives anything. If the second accepts, the first receives the amount they proposed while the second player gets the rest. This game is often used to study aversion to inequity or fairness behavior and its neural basis (Sanfey et al., 2003). There are many other experimental games, some played simultaneously, others repeatedly, that explore reputation effects.
Some of these economic games have been tested in children, either using behavior alone or combined with EEG. For example, one study of event-related potentials (ERPs) studied preschool children while they watched equal and unequal resource distributions (Cowell et al., 2019). The children were given a limited number of candies to distribute to other children who varied in terms of merit, wealth, and need. The ERPs showed early differentiation between equal distributions and any type of distribution that deviates from equality reflected by a greater frontal negativity. Extremely unequal distributions were also distinguished from slightly unequal distributions by a later evoked response (400−800 ms). Furthermore, differences in the magnitude of this late positive potential (LPP) predicted the distribution of third-party contextual resources taking into account the wealth and merit of the recipient. Consistent with a developmental integration of context into social preferences, another EEG study in 5-year-old children documented a marked difference in both an early (P2) ERP waveform with greater positivity for inequitable versus equitable distributions (to poor or wealthy recipients) and a later LPP difference for equitable versus inequitable distributions, which was also predictive of harsher evaluations of the distributions (Pletti & Paulus, 2020). Together, these behavioral and developmental studies indicate that conceptions of fairness develop in early and middle childhood.
Neurocomputational mechanisms involved in group decision-making
Recent fMRI studies have used more complex economic games to characterize the neurocomputational mechanisms engaged in social decision-making. For example, when making judgments in a group, individuals often revise their initial beliefs about the best judgment to make given what others believe (Park et al., 2017). In collective decisions, both the size of groups and the confidence that each member has in their own judgment determine how much a given individual will adapt to the judgment of the group. Judgment adaptation during collective decisions – a fundamental brain mechanism needed for fluid functioning of social organizations – can be accounted for by Bayesian inference computations. At the time of judgment adaptation, individuals trade off the credibility inferred from their own confidence levels against the credibility of social information. The dorsal anterior cingulate cortex (dACC) was found to represent belief updates, while the lateral frontopolar cortex (FPC) monitors the changes in credibility assigned to social information. These results provide a neurocomputational understanding of how individuals benefit both from the wisdom of larger groups and from their own confidence (Park et al., 2017).
A mechanistic foundation for understanding group decision-making, combining Bayesian models of social interactions and model-based fMRI (Khalvati et al., 2019; Park et al., 2019) has been established. When making decisions in groups, the outcome of one’s decision often depends on the decisions of others, and there is a tradeoff between short-term incentives for an individual and long-term incentives for groups. The ventromedial prefrontal cortex encodes immediate expected rewards as individual utility while the lateral frontopolar cortex encodes group utility (i.e., pending rewards of alternative strategies beneficial for the group). When it is required to change one’s strategy, these brain regions exhibit changes in functional interactions with brain regions engaged in switching strategies. Our findings provide a neurocomputational account of how the brain dynamically computes effective strategies to make adaptive collective decisions.
Representing intentions of others and ‘theory of mind’ areas in the human brain
To make decisions in a social context, humans have to predict the behavior of others, an ability that is thought to rely on a model of other minds known as ‘theory of mind’. Key brain regions involved in representing information with respect to other individuals include the Medial Prefrontal Cortex (MPFC) and Temporo-Parietal Junction (TPJ). These areas are often implicated in mentalizing, detecting the beliefs of others, or signaling decision variables concerning another individual (see Figure 10). We have shown that a Bayesian model based on partially observable Markov decision processes outperforms existing models in quantitatively predicting human behavior and outcomes of group interactions when the number of people one simultaneously interacts with is large and actions are anonymous (Khalvati et al., 2019). Our results suggest that in decision-making tasks involving large groups with anonymous members, humans use Bayesian inference to model the “mind of the group,” making predictions of others’ decisions while also simulating the effects of their own actions on the group’s dynamics in the future.
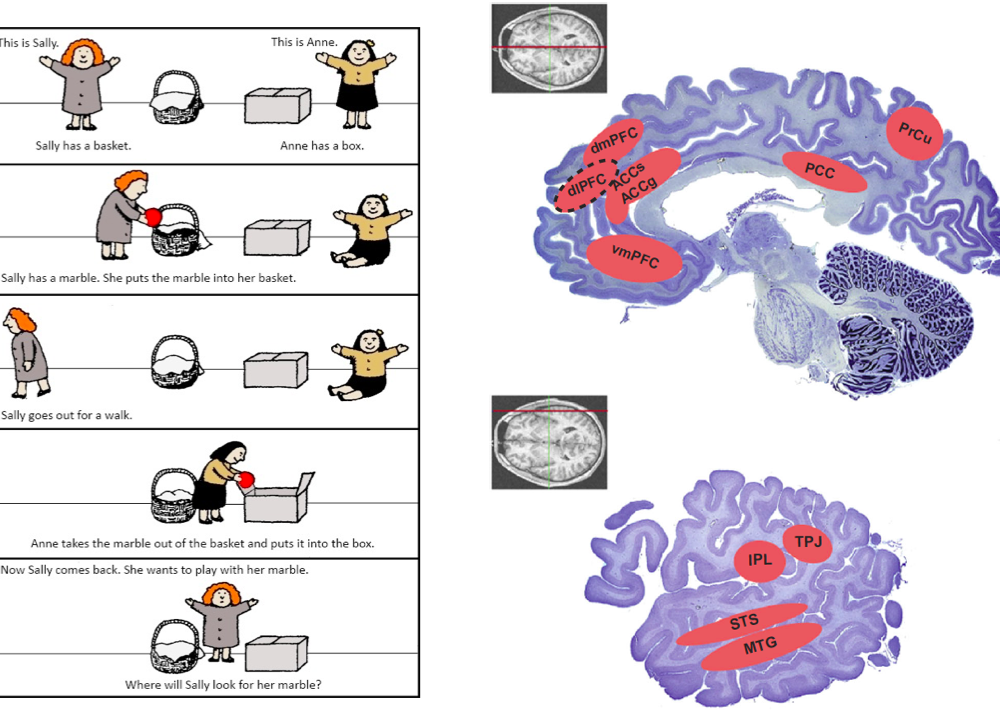
Figure 10. Left: depiction of the classical ‘Sally and Anne’ task used for assessing Theory of Mind (TOM) abilities (Baron-Cohen et al., 1985). Sally takes a marble and hides it in her basket. She then “leaves” the room and goes for a walk. While she is away, Anne takes the marble out of Sally’s basket and puts it in her own box. Sally is then reintroduced, and the child is asked the key question: “Where will Sally look for her marble?” Right: key brain regions involved in representing information with respect to another individual. These areas are often implicated in mentalizing, detecting the beliefs of others, or signaling decision variables concerning another individual (Joiner et al., 2017).
Conclusion
Recent advances in decision neuroscience/neuroeconomics, social psychology and developmental neuroscience allow researchers to identify the neural basis of social decision-making. This interdisciplinary field studies how social stimuli such as faces are recognized, how social learning and complex social interactions, such as group decision-making or attribution of intentions to others (theory of mind) are represented in the brain. Although most of the research hitherto concerns the adult brain, much research remains to be conducted to determine how the social brain develops in infancy, childhood and adolescence.
References
Bao, P., She, L., McGill, M., & Tsao, D. Y. (2020). A map of object space in primate inferotemporal cortex. Nature, 583(7814), 103–108. https://doi.org/10.1038/s41586-020-2350-5
Baron-Cohen, S., Leslie, A. M., & Frith, U. (1985). Does the autistic child have a “theory of mind” ? Cognition, 21(1), 37–46. https://doi.org/10.1016/0010-0277(85)90022-8
Behrens, T. E. J., Hunt, L. T., & Rushworth, M. F. S. (2009). The Computation of Social Behavior. Science, 324(5931), 1160–1164. https://doi.org/10.1126/science.1169694
Bickart, K. C., Wright, C. I., Dautoff, R. J., Dickerson, B. C., & Barrett, L. F. (2011). Amygdala volume and social network size in humans. Nature Neuroscience, 14(2), 163–164. https://doi.org/10.1038/nn.2724
Cavadini, T., Richard, S., Dalla-Libera, N., & Gentaz, E. (2021). Emotion knowledge, social behaviour and locomotor activity predict the mathematic performance in 706 preschool children. Scientific Reports, 11(1), 14399. https://doi.org/10.1038/s41598-021-93706-7
Cowell, J. M., Sommerville, J. A., & Decety, J. (2019). That’s not fair: Children’s neural computations of fairness and their impact on resource allocation behaviors and judgments. Developmental Psychology, 55(11), 2299–2310. https://doi.org/10.1037/dev0000813
Dunbar, R. I. M. (1992). Neocortex size as a constraint on group size in primates. Journal of Human Evolution, 22(6), 469–493. https://doi.org/10.1016/0047-2484(92)90081-J
Dunbar, R. I. M., & Shultz, S. (2007). Evolution in the Social Brain. Science. https://doi.org/10.1126/science.1145463
Gweon, H. (2021). Inferential social learning: Cognitive foundations of human social learning and teaching. Trends in Cognitive Sciences, 25(10), 896–910. https://doi.org/10.1016/j.tics.2021.07.008
Hesse, J. K., & Tsao, D. Y. (2020). The macaque face patch system: A turtle’s underbelly for the brain. Nature Reviews Neuroscience, 21(12), 695–716. https://doi.org/10.1038/s41583-020-00393-w
Izard, C., Fine, S., Schultz, D., Mostow, A., Ackerman, B., & Youngstrom, E. (2001). Emotion Knowledge as a Predictor of Social Behavior and Academic Competence in Children at Risk. Psychological Science, 12(1), 18–23. https://doi.org/10.1111/1467-9280.00304
Joiner, J., Piva, M., Turrin, C., & Chang, S. W. C. (2017). Social learning through prediction error in the brain. Npj Science of Learning, 2(1), 8. https://doi.org/10.1038/s41539-017-0009-2
Kane, A., Lloyd, J., Zaffran, M., Simonsen, L., & Kane, M. (1999). Transmission of hepatitis B, hepatitis C and human immunodeficiency viruses through unsafe injections in the developing world: Model-based regional estimates. Bulletin of the World Health Organization, 77(10), 801–807.
Kanwisher, N., McDermott, J., & Chun, M. M. (1997). The Fusiform Face Area: A Module in Human Extrastriate Cortex Specialized for Face Perception. Journal of Neuroscience, 17(11), 4302–4311. https://doi.org/10.1523/JNEUROSCI.17-11-04302.1997
Khalvati, K., Park, S. A., Mirbagheri, S., Philippe, R., Sestito, M., Dreher, J.-C., & Rao, R. P. N. (2019). Modeling other minds: Bayesian inference explains human choices in group decision-making. Science Advances, 5(11), eaax8783. https://doi.org/10.1126/sciadv.aax8783
Kravitz, D. J., Saleem, K. S., Baker, C. I., & Mishkin, M. (2011). A new neural framework for visuospatial processing. Nature Reviews Neuroscience, 12(4), 217–230. https://doi.org/10.1038/nrn3008
Lecce, S., Caputi, M., Pagnin, A., & Banerjee, R. (2017). Theory of mind and school achievement: The mediating role of social competence. Cognitive Development, 44, 85–97. https://doi.org/10.1016/j.cogdev.2017.08.010
Livingstone, M. S., Vincent, J. L., Arcaro, M. J., Srihasam, K., Schade, P. F., & Savage, T. (2017). Development of the macaque face-patch system. Nature Communications, 8(1), 14897. https://doi.org/10.1038/ncomms14897
Lockwood, P. L., Apps, M. A. J., & Chang, S. W. C. (2020). Is There a ‘Social’ Brain? Implementations and Algorithms. Trends in Cognitive Sciences, 24(10), 802–813. https://doi.org/10.1016/j.tics.2020.06.011
Mishkin, M., Ungerleider, L. G., & Macko, K. A. (1983). Object vision and spatial vision: Two cortical pathways. Trends in Neurosciences, 6, 414–417. https://doi.org/10.1016/0166-2236(83)90190-X
Olsson, A., Knapska, E., & Lindström, B. (2020). The neural and computational systems of social learning. Nature Reviews Neuroscience, 21(4), 197–212. https://doi.org/10.1038/s41583-020-0276-4
Park, S. A., Goïame, S., O’Connor, D. A., & Dreher, J.-C. (2017). Integration of individual and social information for decision-making in groups of different sizes. PLOS Biology, 15(6), e2001958. https://doi.org/10.1371/journal.pbio.2001958
Park, S. A., Sestito, M., Boorman, E. D., & Dreher, J.-C. (2019). Neural computations underlying strategic social decision-making in groups. Nature Communications, 10(1), 5287. https://doi.org/10.1038/s41467-019-12937-5
Pitcher, D., & Ungerleider, L. G. (2021). Evidence for a Third Visual Pathway Specialized for Social Perception. Trends in Cognitive Sciences, 25(2), 100–110. https://doi.org/10.1016/j.tics.2020.11.006
Pletti, C., & Paulus, M. (2020). Neural processing of equitable and inequitable distributions in 5-year-old children. Social Neuroscience, 15(5), 584–599. https://doi.org/10.1080/17470919.2020.1816578
Qu, C., Bénistant, J., & Dreher, J.-C. (2022). Neurocomputational mechanisms engaged in moral choices and moral learning. Neuroscience & Biobehavioral Reviews, 132, 50–60. https://doi.org/10.1016/j.neubiorev.2021.11.023
Sallet, J., Mars, R. B., Noonan, M. P., Andersson, J. L., O’Reilly, J. X., Jbabdi, S., Croxson, P. L., Jenkinson, M., Miller, K. L., & Rushworth, M. F. S. (2011). Social Network Size Affects Neural Circuits in Macaques. Science, 334(6056), 697–700. https://doi.org/10.1126/science.1210027
Sanfey, A. G., Rilling, J. K., Aronson, J. A., Nystrom, L. E., & Cohen, J. D. (2003). The Neural Basis of Economic Decision-Making in the Ultimatum Game. Science, 300(5626), 1755–1758. https://doi.org/10.1126/science.1082976
Semendeferi, K., Lu, A., Schenker, N., & Damasio, H. (2002). Humans and great apes share a large frontal cortex. Nature Neuroscience, 5(3), 272–276. https://doi.org/10.1038/nn814
Teffer, K., & Semendeferi, K. (2012). Chapter 9 – Human prefrontal cortex: Evolution, development, and pathology. In M. A. Hofman & D. Falk (Eds.), Progress in Brain Research (Vol. 195, pp. 191–218). Elsevier. https://doi.org/10.1016/B978-0-444-53860-4.00009-X
Torres, M. M., Domitrovich, C. E., & Bierman, K. L. (2015). Preschool interpersonal relationships predict kindergarten achievement: Mediated by gains in emotion knowledge. Journal of Applied Developmental Psychology, 39, 44–52. https://doi.org/10.1016/j.appdev.2015.04.008
Ursache, A., Kiely Gouley, K., Dawson-McClure, S., Barajas-Gonzalez, R. G., Calzada, E. J., Goldfeld, K. S., & Brotman, L. M. (2020). Early Emotion Knowledge and Later Academic Achievement Among Children of Color in Historically Disinvested Neighborhoods. Child Development, 91(6), e1249–e1266. https://doi.org/10.1111/cdev.13432
van Dijk, E., & De Dreu, C. K. W. (2021). Experimental Games and Social Decision Making. Annual Review of Psychology, 72(1), 415–438. https://doi.org/10.1146/annurev-psych-081420-110718
Voltmer, K., & von Salisch, M. (2017). Three meta-analyses of children’s emotion knowledge and their school success. Learning and Individual Differences, 59, 107–118. https://doi.org/10.1016/j.lindif.2017.08.006
Waiden, T. A., & Field, T. M. (1990). Preschool children’s social competence and production and discrimination of affective expressions. British Journal of Developmental Psychology, 8(1), 65–76. https://doi.org/10.1111/j.2044-835X.1990.tb00822.x