Co-occurring disorders of learning: Why they matter for practice and research in educational neuroscience
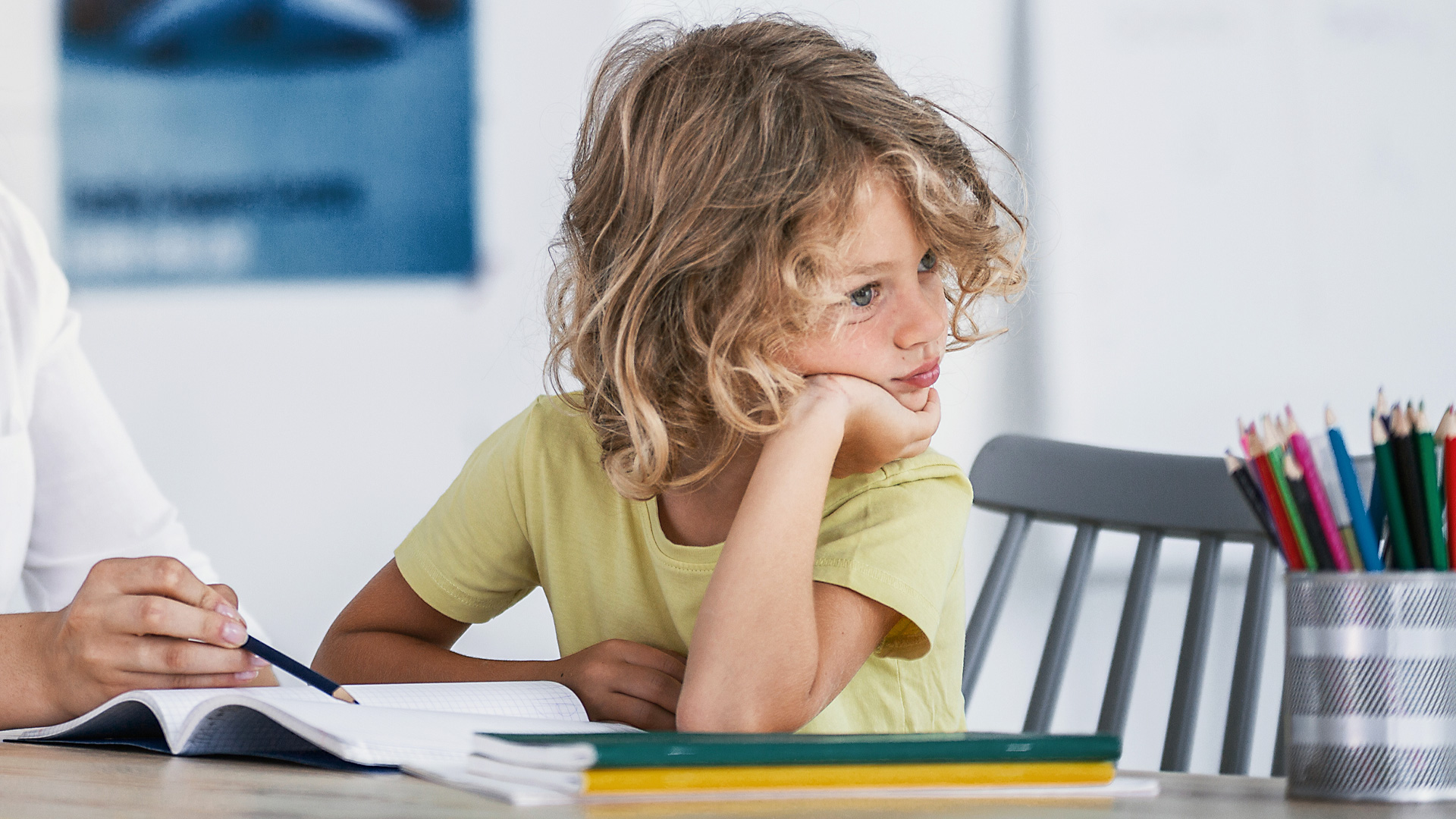
Executive summary
- Comorbidity is defined as when two or more conditions occur in the same individual at the same time.
- The high comorbidity of many conditions that compromise children’s learning, such as dyslexia (affects reading), dyscalculia (affects mathematics), attention deficit hyperactivity disorder (affects executive function), and language impairment (affects speech and language), is enough to threaten the assumption that their underlying causes are unrelated.
- Children with co-occurring disorders may have more severe difficulties and more complex educational needs than do other children.
- A shift in screening and assessment practice from a focus on disorder categories to broader dimensional approaches will advance how children learning disorders are identified, characterised and followed through development.
Human beings are typically very good at separating the things that we encounter in our environments into conceptual categories. Plato likened the process of deriving valid categories from our observations as ‘carving nature at its joints’1. Such categorisation of things or of phenomena can involve making both conscious and unconscious inferences about their characteristics, and this normally aids us in structuring and simplifying our observations and understanding of the world around us. Our best theories about the organisation of the world help us to make valid distinctions between natural kinds of things, as Plato had surmised. When misapplied, however, a reliance on categorical thinking can result in failures of attribution, such as in drawing unjustified inferences about the characteristics of individuals within the categories we have derived. For example, we tend to perceive variability in the features we observe across individuals (for e.g., psychological traits) as being systematically smaller within a perceived category, compared to that which occurs across categories, even when available evidence suggests otherwise. Ultimately this, and other types of attribution error, threaten both the validity of the categorical judgements we make and decisions about what we do with them, as neither the prototypical nor the average member of a category necessarily represents any individual member within that notional grouping. For example, the diagnostic label ‘dyslexia’ may be useful in describing children who are falling behind their peers in attaining benchmarks of reading and literacy achievement, but this unidimensional label does not sufficiently capture the variability across children in either the underlying causes or in the behavioural manifestations of their reading disability at the individual level2-5. Over-reliance on categorical labels may also encourage ‘one-size, fits-all’ approaches to decisions about approaches to intervention, which may ultimately underperform more personalised remedial approaches based on a more detailed and specific understanding of individual needs.
This brief focuses on a topic within the science of learning in which categorical thinking has dominated both theory and practice since its inception, namely the conventional medico-psychiatric approach to how disorders of mental health and cognition are characterised and diagnosed. Using the diagnostic labels that we traditionally apply to children’s specific learning disorders as a platform, this brief provides a summary of how evidence about their co-occurring symptom dimensions and probable underlying causes, threatens historical beliefs about their presumed status as specific and distinct diagnostic entities. This debate is important, because our understanding of the nature of disorder has widespread implications for both the provision and the type of diagnostic, intervention and service provision offered to those children affected and their families. In part, this issue arises from the contrasting roles that assessment practice and research apply toward identifying and validating disorder types that are putatively different with respect to both their surface properties and their underlying mechanisms. Current assessment practice is mainly purposed for identifying features of disorders at the individual level. While this provides a useful approach for recognising children who may benefit from additional or alternative support for their learning, it is a process conceptually different from that involved in the testing and validation of disorder taxonomies, based on characterisation of the mechanisms and symptoms that can be used to distinguish between disorders that are predicted (and very often presumed) to be causally independent.
The diagnostic co-occurrence of neurodevelopmental disorders of learning is high
By the current diagnostic conventions imbued in the Diagnostic and Statistical Manual of Mental Disorders (DSM-V)6 and the International Classification of Disease (ICD-10)7, ‘specific learning disorders’ ‘specific developmental disorders of scholastic skills’ in ICD-10) are identified by a child’s persistent difficulties in learning and using academic skills, with symptoms specific to at least one of the following domains: reading accuracy and fluency, reading comprehension, spelling, written expression, numerical calculation or mathematical reasoning. These observed deficits in particular domains of learning achievement are characterised by their onsets in childhood, at which measured levels of attainment are at levels below that expected for a given chronological age, and which persist over time. Moreover, the difficulties are not better explained by the presence (or absence) of other obvious causes, either those that are internal to the individual (i.e., sensory or overt neurological factors) or environmental (i.e., lack of access to educational resources). These disorders comprise some of the most frequently diagnosed conditions of childhood, and with highly variable prevalence rates in the population. Depending upon how they have been sampled and the exact criteria applied within relevant assessment practice, the estimated prevalence of the most commonly diagnosed specific learning disorders – those in reading, mathematics, language or attention – each hovers at between 3 and 10 percent of the population8-14. In context, these high prevalence rates for each individual disorder reflect that, on average, there is at least one child in every school classroom with at least one of these conditions. Often persisting long beyond childhood, these developmental disorders of learning have long-term impacts on individual quality of life and incur high levels of societal cost15, particularly if they are not addressed through targeted interventions at the earliest ages possible.
Contemporary research is beginning to show that developmental disorders of childhood, including specific learning disorders, have high rates of co-occurrence within the same individuals16,17, and that this occurs at a frequency much higher than would be expected through their aggregation by random processes. Indeed, a large proportion of individuals with a diagnosis of any disorder identified within the current DSM/ICD diagnostic structures would also be predicted to satisfy the criteria for a diagnosis of at least one additional disorder at some point during their lifetime18. For disorders of learning, high rates of co-occurrence, and particularly comorbidity within common timeframes of development16,17, provide evidence that challenges pre-existing assumptions about their status as discrete diagnostic entities. Comorbidity also raises important practical issues for how the educational needs of these children with complex individual patterns of difficulty can best be identified and addressed through targeted interventions18. For example, a child with reading disorder (dyslexia), may experience limitations in phonological language and working memory skills severe enough to impede their learning of the mappings that govern the relationships between letters and speech sounds that are crucial for sounding out novel words. If this child also has difficulties typically associated with ADHD, they may experience additional challenges associated with reduced attentional vigilance and control and through the negative impacts of associated behavioural difficulties on their learning (for e.g., school exclusion). Together, these factors would be expected to compound the severity of any learning difficulties, manifest through their combined or interactive effects. Children with co- or multi-morbidities may therefore experience greater interference with their learning, have poorer academic outcomes, and place greater demands on the resourcing available from the educational and tertiary services that are in place to support them18,19.
Diagnostic categories: The DSM/ICD approach
The high co-occurrence rates between disorders challenges existing conceptions of their status as independent, categorical entities. The dominant theoretical perspective of how more than one disorder manifests at an individual level has been driven by a nearly 150-year history of psychiatric practice, derived initially from that applied for the understanding and classification of diseases, and subsequently translated to the diagnosis of human psychiatric and mental health conditions. This approach is fundamentally based on the principles of differential diagnosis, the identifying of and linking between specific sets of symptoms to unique disorder categories. In medical classification systems – nosology – ‘comorbidity’ is used to describe the situation when diseases/disorders that are etiologically independent, both in their causes and their symptoms, co-occur within the same individual at the same time.
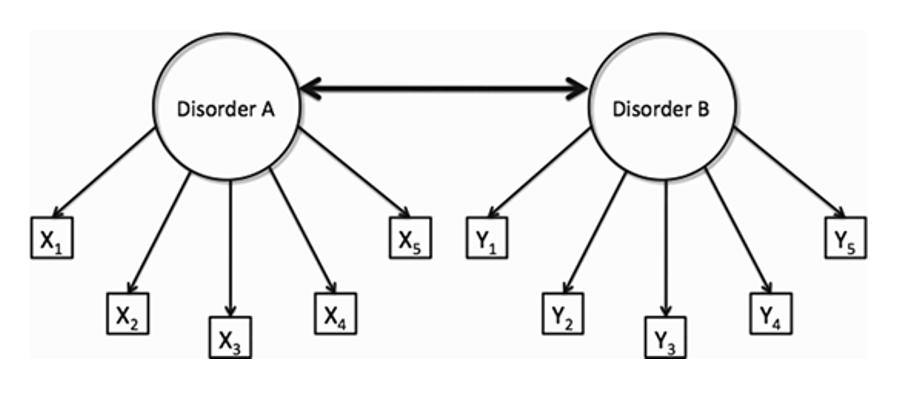
Figure 1. Conceptual schematic of a classification system based on the dual premises of disorder independence and of differential diagnosis. Two hypothetical disorders (A and B) are each associated with a unique set of symptoms (x or y) that are independent and non-overlapping, either with each other or with those of other conditions. By this view, co-occurrence of two or more of these disorders (bidirectional arrow) manifests predominantly at random.
Figure 1 depicts the dominant mindset of traditional diagnostic practice, namely, that disorders are implicitly presumed to be causally and etiologically independent, diagnosed and differentiated from another by their unique and non-overlapping symptom sets. From these models, the frequency of more than one disorder co-occurring within the same individual can be estimated probabilistically, based on the assumption of their aggregation strictly by random processes, and their estimated individual prevalence rates in the population. For example, consider two hypothetical disorders A and B, each affecting 5% of the overall population. By the medical model, the prevalence of their co-occurrence should approach 0.25% or between two and three individuals per one thousand within the broader population. These prevalence estimates can be used to frame predictions about alternative models for why disorders co-occur, for example, the extent to which they share underlying risk factors, rather than resulting from independent causes. For pediatric developmental disorders, the empirical evidence on their nature in this respect is clear. Irrespective of whether or not the disorders in question are predicted to manifest in the same (homotypic) or different (heterotypic)20 cognitive domains (for e.g., within language or across both language and attention), the observed diagnostic overlap is substantially higher than that which would be predicted if the disorders were indeed independent, and to such an extent that the presence of overlap appears to represent much more the typical case, rather than the exception21,22. This high incidence of overlap extends beyond the developmental disorders of childhood to include other diagnostic categories, for example, those used to describe mental illness, such that nearly half of all persons who meet the criteria for any one disorder typically meet the diagnostic criteria for at least one additional diagnosis23-25. [i]
Figure 2 provides a representative depiction of the overlaps in co-occurrence for some of the most prevalent developmental disorders that impact upon children’s learning in educational environments. From 15% to 40% of persons with dyslexia also satisfy the criteria for a diagnosis of ADHD26-27. The observed diagnostic overlap between dyscalculia and dyslexia ranges between 37% and 64% 28,29. Correspondence between presumed heterotypic impairments, for example between language disorder and mathematics disorder30 and between dyscalculia and ADHD31, is similarly high and suggests that the overlaps aren’t simply driven by overlaps in their diagnostic criteriacf. 32.
[i] Part of this difficulty also emanates from the content of the instruments used in assessment practice, which maybe ill-constructed for the purpose of achieving a differential diagnosis. A comprehensive analysis has revealed widespread inconsistencies in the types of symptoms assessed for individual disorders as well as large overlaps in the symptoms described across a range of measures that are proposed to yield disorder-specific information32.
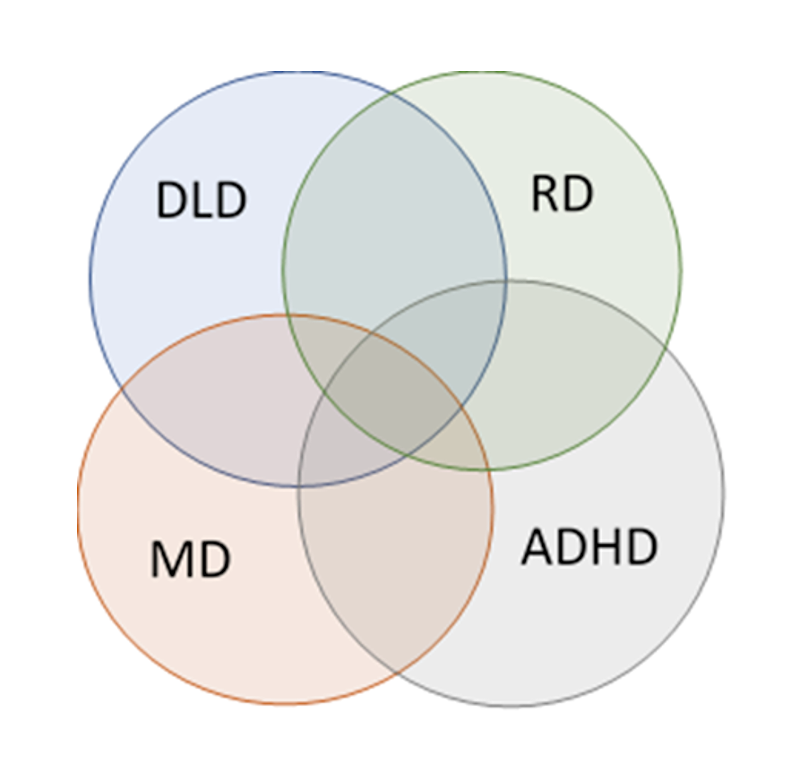
Figure 2. Diagram showing the high degrees of overlap between four of the most prevalent disorders that impact negatively upon learning in childhood. RD: reading disorder, DLD: developmental language disorder; ADHD: attention-deficit hyperactivity- disorder; MD: mathematics disorder. The extent of overlaps is based on a hypothetical overlap between pairs of disorders of approximately one-third.
Comorbidity challenges a science of learning difficulties based on differential diagnosis
The empirical evidence regarding developmental disorders of childhood suggests that more than one diagnosis will often be necessary to describe the symptom set that characterises an individual. Our emerging understanding of developmental disorders of learning and cognition is that they should no longer simply be assumed to be invariant, discrete disorders, with presumed independent and specific causes, as the presence of the specific diagnostic labels typically given to individual children may imply. We therefore need new models that sufficiently capture the reality of this diagnostic complexity. Alternatives to categorical perspectives on developmental disorders are now beginning to find traction and are promoting a shift from thinking about disorders in strict terms connoting discrete diagnostic entities which are invariant in their symptom sets, toward broader and more integrative transdiagnostic perspectives33-36. This paradigm shift when fully translated into diagnostic practice, promises significant changes in the way assessment and research practice will be conducted, streamlining diagnostic pathways, and enabling intervention and treatment approaches that are tailored more to the needs of individuals rather than to groups.
To date, disorders of learning achievement are most often diagnosed on the basis of behavioural features, usually obtained via some combination of standardised testing and clinically-oriented observation. Standardised tests are used to benchmark an individual’s profile of scores on individual tests of achievement or ability to the distribution of scores obtained for a reference population of children of the same chronological age. The child’s performance on a given measure is reported as a standard score, which represents the distance of the individual score from that of the population average for the appropriate age group. These scores can also be reported as percentiles, which provide an estimation of the percentage of children in the population who would score lower than or equal to the individual child in question on a particular standardised measure. In current assessment practice, rather arbitrary cut-off points are imposed by convention on these continuous measurement scales to create pseudo-categories of diagnoses. For example, a typical diagnosis of dyslexia may require scores on one or more constituent measures of reading and component skills to be at or below a standard score of 85 (~16th percentile for a standard score described by a population mean of 100 and a standard deviation of 15). Children who scored above this statistical cut-off would not typically receive a formal diagnosis, whereas children who scored at or below the cut-off would. This categorical logic becomes particularly problematic at the cut-off point, as score differences with little to no relevance to the severity of a child’s measured difficulties (for e.g, the functional difference between a standard score of 85 versus that of 86) can make a big difference to the probability of whether or not they receive a diagnosis and the additional support and resource that often comes with it.
Assessment based on behavioural features suffers from additional limitations, in that neither objective (e.g, standard scores) nor subjective (e.g, clinical observations) descriptions of behaviour can describe or predict the underlying causes of disorder sufficiently. Surface descriptions of observable behaviour are logically separate from the identification of their underlying causes. For example, a reading impairment identified in two children, each with a standard score of 80 on a standardised measure of reading, could be predicted to result from one or more of a set of underlying mechanisms, which need not be identical in their presence or impact for each child. To further illustrate this point, consider our study37 that compared children with and without reading difficulties, identified by their profile of scores on standardised tests of achievement. Across three ‘deficit’ groups of children, stratified by their relative ability to name pseudowords (e.g. ‘torlep’) and exception words (e.g. ‘yacht’), the average reading ability of each of the groups with reading impairments was well below the average, but there was actually little difference between the average magnitude of the (lower) reading scores across the groups. However, when the groups were compared to different comparison groups, comprised either of children of the same chronological age or of younger, typically developing readers matched to the deficit groups on reading level, the patterns of data suggested that the groups arrived at their reading (dis)ability through different developmental mechanisms (trajectories). One deficit pattern presented as a pattern of atypical development, in which children’s reading achievement would be predicted to remain incommensurate with age expectancies over time, whereas the second pattern observed a more simple pattern of developmental lag, which resembled a profile of a younger, typically developing reader. The implication of this result is that children with similar profiles of (low) scores on standardised behavioural measures of reading achievement, may be described diagnostically in terms that would imply that similar developmental mechanisms were at play, when in fact the evidence suggests that they arrived at this profile through developmental trajectories that were different. Appreciating the potentially different developmental trajectories of these groups may also impact upon the choice of intervention strategy applied, toward improving reading scores in these groups. For example, a pattern of comparatively simple developmental delay might be predicted to be remediated through increased developmental maturity and via regular exposure to mainstream classroom learning environments. In contrast, a pattern of atypical development might be predicted to require more intensive and tailored support to promote the sustained reading achievement of the child.
Data such as these support the existence of distinct patterns of reading impairment that is characterised by more heterogeneous patterns of symptoms, in which there exists a broad constellation of symptoms that are also variably present across individuals5,38-40. In other words, there is a range of routes through which an individual may become a poor reader or a poor mathematician, but many of these routes are insufficiently captured by current diagnostic labels. Applied to disorders of learning more broadly, research evidence now suggests the near ubiquity of variability in both the surface symptoms and in the underlying causes of learning disorders5, and this poses significant difficulties for their conceptualisation as discrete diagnostic entities.
Toward a science of learning disabilities based on dimensional risk factors
The characteristic symptoms of learning disabilities typically vary widely within existing categories and also substantially overlap between them. Without sufficient knowledge of their shared and non-shared mechanisms, however, we cannot be comfortable in assuming that individuals who meet current diagnostic criteria for more than one disorder actually have unrelated conditions. The potential for overlapping causes and symptoms of learning disorders provides both conceptual and practical barriers to understanding their nature and ultimately for designing and choosing effective intervention pathways based on the needs of individuals rather than categories.
A key distinction between the parallel aims of assessment and nosology is the reliance of current assessment practice on behavioural measures, the observable manifestation of internal mechanisms, at different levels of measurement. A developing taxonomic approach to understanding how disorders manifest and develop in children now incorporates a range of convergent neuroscience methods, from genetics, through brain imaging, to fine-grained analyses of cognition, in order to supplement surface level descriptions of observable behaviour. A schematic example of such a taxonomic approach is shown in Figure 3. The figure41 is a significant adaptation of a causal framework of cognitive developmental disorders, first proposed by Morton and Frith42, in which potential risk factors for the disorder can be addressed at multiple levels of analysis, across genes, brains, cognition and behaviour. In contrast to the categorical diagnostic approach represented by Figure 1, this dimensional framework provides a significant step change in guiding research toward understanding the similarities and differences in surface features of disorders and their co-occurrence, whilst appreciating that underlying risk factors can be either single or multiple, and either unique or shared across different disorder clusters. Moreover, it captures emerging empirical evidence that the underlying risk factors across multiple disorders of development, across levels of analysis across genes43,44, brains45-47, and behaviour48,49, act to modify risk for disorders in a continuous manner in everyone, rather than through mechanisms that are unique to specific disorders and not to the general population. Disorders and their comorbidities can therefore be conceptualised as occurring when a threshold of risk is reached through the accumulation of multiple underlying mechanisms, each with the potential to vary in potency within and across individuals50-51.
Crucial to the emerging multi-dimensional model is that risk factors between different levels of analysis can have relationships that are both many to one (for e.g., many genes impacting common neurobiological targets) and one to many (for e.g., the impact of one cognitive domain on multiple behaviour patterns). For example, a common risk factor at the cognitive level, for example in the allocation and control of attention (for e.g., cog 2), can contribute to risk for both ADHD (beh 2 ), reading disorder (beh 3) and autism (beh 1), whereas a deficit in phonological decoding of written language (for e.g., cog 3) might ultimately be shown to be specific to the reading disorder.
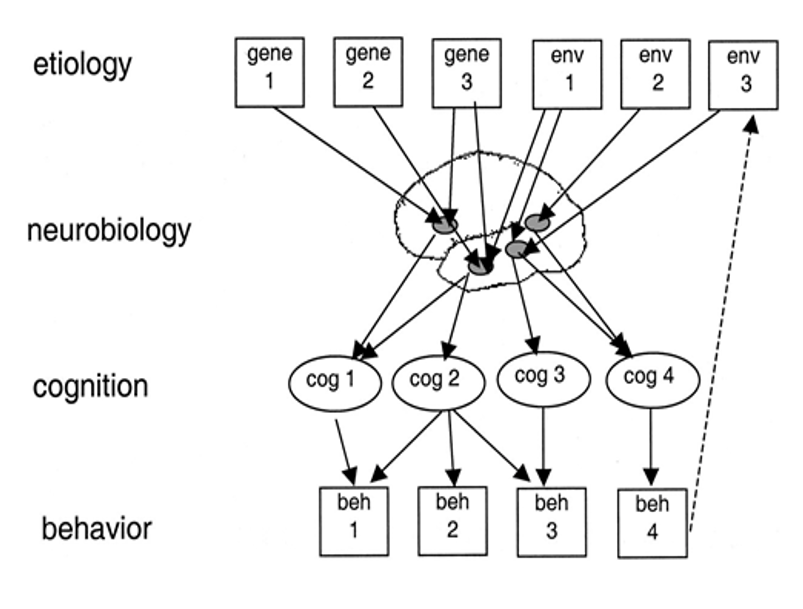
Figure 3. A multilevel framework for the causes of developmental disorders observed behaviour patterns or diagnostic entities (beh). The underlying causes of manifest behaviour can be described as resulting from a cascade of effects, with origins in different levels, from genes and environments, neurobiology, to cognition. Figure reproduced from Figure 2 of Bishop, D. V. M., & Snowling, M. J. (2004). Developmental Dyslexia and Specific Language Impairment: Same or Different? Psychological Bulletin, 130(6), 858–886. https://doi.org/10.1037/0033-2909.130.6.858, [ref. 41], and downloaded from https://images.app.goo.gl/L9K4GY9sfmhauRzV8
Traditional conceptions of disorder-independence and explanations for their comorbidity present challenges both for current assessment practice, and for the theoretical understanding of disorder taxonomies. Education systems are positioned at the intersection of these different facets within the science of learning. Current assessment practice is particularly useful for identifying children who show features of disability at the individual level and for whom interventions may be of benefit, but it is much less useful for deriving groups and validating clusters of cases with the same underlying cognitive and biological causes. Such poor taxonomic understanding of disorder modifies the way that we think about and define disorder categories, and ultimately this contributes to children receiving different diagnoses for the same sets of symptoms, particularly if they were captured by different practitioners in different diagnostic referral route
The dimensional systems perspective developed by the NIMH Research Domains Framework34,52 is challenging conventional thinking about the relevance and impact of disorder comorbidities within the science of learning. Within this perspective, disorders are conceptualised less as discrete categories, and more as continuous dimensions where both the behaviours associated with the disorder and the underlying mechanisms involved can vary across the full range of performance or symptom severity for each individual. Appreciating this potential heterogeneity, both within and across groups of individuals with learning difficulties, has significant implications for educational practice, including the potential to offer interventions that are selected and refined for the needs of individuals rather than simply for groups with the same diagnostic labels.
Although not yet implemented in widespread assessment practice, an emerging multilevel understanding of diagnostic complexity provides a pragmatic and potentially powerful alternative to the collapsing of individual behaviour patterns into a few, ill-formed diagnostic categories. Current diagnostic systems therefore risk the silo of relying upon single diagnoses, which are susceptible to incompletely describing complex disorders, and thereby introducing delays to families accessing the alternative and additional diagnoses that are often needed by their children. Inefficiencies in these systems also increase both financial and opportunity costs to families and other stakeholder groups, creating stress and ultimately delaying the onset of effective support for children at the earliest ages possible. Considerations of alternative, single pathway assessment systems may be better suited to assess individual variability and diagnostic complexity as a starting point, and ultimately offer an advancement of how learning difficulties are identified, characterised and followed over time.
References
- Plato in Twelve Volumes, Vol. 9 translated by Harold N. Fowler. Harvard University Press; William Heinemann Ltd. 1925. Plato, Phaedrus, 265e.
- McArthur, G., et al. (2013). Getting to grips with the heterogeneity of developmental dyslexia. Cognitive Neuropsychology, 30, 1–24.
- Castles, A., & Coltheart, M. . (1993). Varieties of developmental dyslexia. Cognition, 47, 149–180.
- Carioti, D., et al. (2020). Orthographic depth and developmental dyslexia: a meta-analytic study. Annals of Dyslexia, 71, 399–438. https://doi.org/10.1007/s11881-021-00226-0
- Pennington, B.F. (2006). From single to multiple deficit models of developmental disorders. Cognition, 101, 385–413.
- American Psychiatric Association. (2013). Diagnostic and statistical manual of mental disorders (fifth addition). American Psychiatric Association.
- World Health Organization. (2004). ICD-10: international statistical classification of diseases and related health problems: tenth revision, 2nd ed. World Health Organization.
- Peterson, R.L., & Pennington, B.F. (2012). Developmental dyslexia. Lancet, 379, 1997–2007.
- Stothard, S.E., & Hulme, C. (1992). A comparison of reading comprehension and decoding difficulties in children. J Child Psychol Psychiatry, 36, 399–408.
- Catts, H.W., Hogan, T.P., & Fey, M.E. (2003). Subgrouping poor readers on the basis of individual differences in reading-related abilities. J Learn Disabil, 36, 151–64.
- Laasonen, M., et al. (2018). Understanding developmental language disorder – The Helsinki longitudinal SLI study (HelSLI): A study protocol. BMC Psychol, 6, 24. https://doi.org/10.1186/s40359-018-0222-7
- Morsanyi, K., van Bers, B.M.C.W., McCormack, T., & McGourty J. (2018). The prevalence of specific learning disorder in mathematics and comorbidity with other developmental disorders in primary school-age children. Br J Psychol, 109, 917–940.
- Geary, D.C. (1993). Mathematical disabilities: Cognitive, neuropsychological, and genetic components. Psychol Bull, 114, 345–362.
- Thomas, R., Sanders, S., Doust, J., Beller, E., & Glasziou, P. (2015). Prevalence of attention-deficit/hyperactivity disorder: A systematic review and meta-analysis. Pediatrics, 135, 994–1001.
- Lamsal, R., & Zwicker, J.D. (2017). Economic evaluation of interventions for children with neurodevelopmental disorders: Opportunities and challenges. Health Econ. Health Policy, 15, 763–772.
- Moll, K., Kunze, S., Neuhoff, N., Brude, J., & Schulte-Körne, G. (2014). Specific learning disorder: Prevalence and gender differences. PLoS ONE, 9, e103537.
- Landerl, & Moll, K. (2010). Comorbidity of learning disorders: prevalence and familial transmission. J Child Psychol Psychiatry, 51, 287–294.
- Valderas, J.M., Starfield, B., Sibbald, B., Salisbury, C., & Roland, M. (2009). Defining comorbidity: implications for understanding health and health services. Ann Fam Med, 7, 357–363.
- Dewey, D. (2018). What Is comorbidity and why does it matter in neurodevelopmental disorders? Curr Dev Disord Rep, 5, 235–242.
- Angold, A., Costello, E.J., & Erkanli, A. (1999). Comorbidity. J Child Psychol Psychiatry, 40, 57–87.
- Kaplan, B.J., Dewey, D.M., Crawford, S.G., & Wilson, B.N. (2001). The term comorbidity is of questionable value in reference to developmental disorders: Data and theory. J Learn Disabil, 34, 555–565.
- Pennington, B.F. (2001). Diagnosing learning disorders: A neuropsychological framework (2nd. ed). Guildford Press.
- Kessler, R.C., et al. (2012). Lifetime co-morbidity of DSM-IV disorders in the US National Comorbidity Survey Replication Adolescent Supplement (NCS-A). Psychol Med, 42, 1997–2010.
- Andrews, G., Slade, T., & Issakidis, C. (2002). Deconstructing current comorbidity: data from the Australian National Survey of Mental Health and Well-Being. Br J Psychiatry, 181, 306–314.
- Bijl, R.V., Ravelli, A., & van Zessen, G. (1998). Prevalence of psychiatric disorder in the general population: results of The Netherlands Mental Health Survey and Incidence Study (NEMESIS). Soc Psychiatry Psychiatr Epidemiol, 33, 587–595.
- DuPaul, G.J., Gormley, M.J., & Laracy, S.D. (2013). Comorbidity of LD and ADHD: Implications of DSM-5 for assessment and treatment. J Learn Disabi, 46, 43–51.
- Willcutt, E.G. & Pennington, B.F. Comorbidity of reading disability and attention-deficit/hyperactivity disorder: differences by gender and subtype. J Learn Disabil., 33, 179–191 (2000).
- Badian, N.A. Arithmetic and non-verbal learning. (1983). In H.R. Myklebust (Ed). Progress in learning disabilities (Vol 5, pp. 235–264). Grune & Stratton.
- Lewis, C., Hitch, G.J., & Walker, P. (1994). The prevalence of specific arithmetic difficulties and specific reading difficulties in 9- to 10-year-old boys and girls. J Child Psychol Psychiatry, 35, 283–292.
- Manor, O., Shalev, R.S., Joseph, A., & Gross-Tsur, V. (2001). Arithmetic skills in kindergarten children with developmental language disorders. Eur J Pediat Neurol, 5, 71–77.
- S.D., Calhoun, S.L., & Crowell, E.W. (2000). Learning disabilities and ADHD: Overlapping spectrum disorders. J Learn Disabil, 33, 417–424.
- Newson, J.J., Hunter, D., & Thiagarajan, T.C. (2020). The heterogeneity of mental health assessment. Frontiers in Psychiatry, 11. https://doi.org/10.1016/j.tine.2019.100115
- Astle, D., Holmes, J., Kievit, R., & Gathercole, S.E. (2021). The transdiagnostic revolution in neurodevelopmental disorders. Pre-print at https://doi.org/10.31234/osf.io/4g3a2
- Cuthbert, B.N., & Insel, T.R. (2013). Toward the future of psychiatric diagnosis: The seven pillars of RDoC. BMC Med, 11. https://doi.org/10.1186/1741-7015-11-126
- Sonuga-Barke, E.J., Cortese, S., Fairchild, G., & Stringaris, A. (2016). Annual research review: Transdiagnostic neuroscience of child and adolescent mental disorders – differentiating decision making in attention-deficit/hyperactivity disorder, conduct disorder, depression, and anxiety. J Child Psychol Psychiatry, 57, 321–349.
- Zhao, Y., & Castellanos, F.X. (2016). Annual research review: Discovery science strategies in studies of the pathophysiology of child and adolescent psychiatric disorders – promises and limitations. J Child Psychol Psychiatry, 57, 421–439.
- Talcott, J.B., Witton, C., & Stein, J.F. (2013). Probing the neurocognitive trajectories of children’s reading skills. Neuropsychologia, 51, 472–481.
- Snowling, M.J. (2008). Specific disorders and broader phenotypes: The case of dyslexia. Q J Exp Psychol, 61, 142–56.
- Menghini, D., et al. (2010). Different underlying neurocognitive deficits in developmental dyslexia: A comparative study. Neuropsychologia, 48, 863–872.
- Ramus, F., et al. (2003). Theories of developmental dyslexia: Insights from a multiple case study of dyslexic adults. Brain, 126, 841–865.
- Bishop, D.V.M., & Snowling, M.J. (2004). Developmental dyslexia and specific language impairment: Same or different? Psychol Bull, 130, 858–886.
- Morton, J., & Frith, U. (1995). Causal modeling: A structural approach to developmental psychopathology. In D. Cicchetti & D. J. Cohen (Eds.), Wiley series on personality processes. Developmental psychopathology, Vol. 1. Theory and methods (p. 357–390). John Wiley & Sons.
- Plomin, R., & Kovas, Y. (2005). Generalist genes and learning disabilities. Psychol Bull, 131, 592–617.
- Cross-Disorder Group of the Psychiatric Genomics Consortium. (2013). Identification of risk loci with shared effects on five major psychiatric disorders: a genome-wide analysis. Lancet, 381, 1371–1379.
- Moreno-De-Luca, A., et al. (2013). Developmental brain dysfunction: Revival and expansion of old concepts based on new genetic evidence. Lancet Neurol, 12, 406–414.
- Krishnan, S., Watkins, K.E., & Bishop, D. (2016). Neurobiological basis of language learning difficulties. Trends Cogn Sci, 20, 701–714.
- Siugzdaite, R., Bathelt, J., Holmes, J., & Astle, D.E. (2020). Transdiagnostic brain mapping in developmental disorders. Biol, 30, 1245–1257.
- Moll, K., Göbel, S.M., Gooch, D., Landerl, K., & Snowling, M.J. (2016). Cognitive risk factors for specific learning disorder: Processing speed, temporal processing, and working memory. Br J Learn Disabil, 49, 1–10.
- Peters, L., & Ansari, D. (2019). Are specific learning disorders truly specific, and are they disorders? Trends Neurosci Educ, 17. https://doi.org/10.1016/j.tine.2019.100115
- Catts, H.W., & Petscher, Y. (2020). A cumulative risk and resilience model of dyslexia. Pre-print at https://doi.org/10.35542/osf.io/g57ph
- Taurines, R., et al. (2010). Developmental comorbidity in attention-deficit/hyperactivity disorder. Atten Def Hyp Disord, 2, 267–289.
- Morris, S.E., & Cuthbert, B.N. (2012). Research domain criteria: Cognitive systems, neural circuits, and dimensions of behavior. Dialogues Clin Neurosci, 14, 29–37.