Lessons for the classroom from neuroeconomics
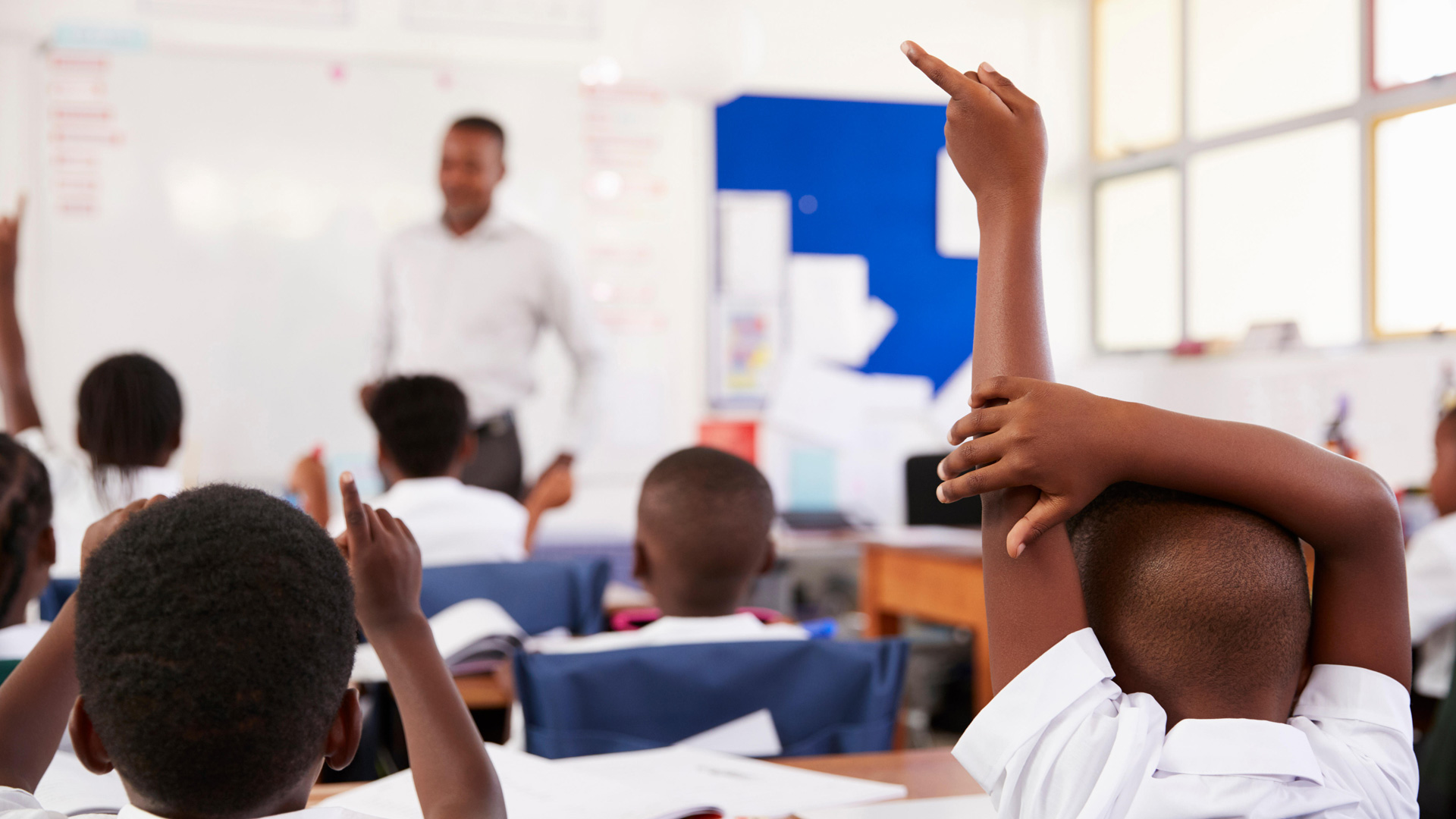
Executive Summary
- Neuroeconomics is a field of research seeking to explain how the brain makes decisions between different options.
- Decision-making occurs in several stages. These include the evaluation of potential options based on costs and benefits and the selection of the option with the highest subjective value.
- The concept of a common currency reflects the fact that our brain has to integrate and compare different types of options in order to choose between them.
- Brain imaging studies in humans have identified a valuation system, involving the ventromedial prefrontal cortex and the ventral striatum, that implements this common currency in the brain to compare between options.
- A classical way of identifying this valuation system involves offering participants a choice between a small immediate reward and a larger delayed reward. This type of choice is known as ‘delay discounting’.
- Delay discounting measures impatience and refers to the empirical finding that both humans and animals value immediate rewards over delayed rewards. Discounting the subjective value of a delayed reward engages the brain valuation system, which involves the ventromedial prefrontal cortex and ventral striatum.
- Cognitive control refers to the ability to override our impulses and make decisions based on our goals. This ability engages the dorsolateral prefrontal-parietal network.
- Adolescents are more likely than adults to opt for immediate smaller rewards rather than larger ones delayed. This greater impatience is reflected in lower connectivity between the dorsolateral prefrontal cortex, part of the cognitive control network, and the ventral striatum, part of the valuation system.
- Higher cognitive control in children predicts future academic achievement.
- Working memory capacity can be increased through training that produces plastic changes in the cognitive control network of the brain.
Neuroeconomics and value-based decision-making
Neuroeconomics is an interdisciplinary field that combines research in neuroscience, behavioral economics and cognitive and social psychology. It seeks to explain how humans and animals choose between different options. Typical topics of research in the field include how individual preferences, value, risk, time preferences and social preferences are learned and reflected in brain activity. The focus of this brief is to introduce important concepts from the field, such as utility and common currency, and to review our current understanding of their neuronal implementation. We will also pinpoint potential implications of this research for education, focusing on the neurodevelopment of cognitive control functions useful for delay discounting and risk taking.
How does the brain make a choice between different options when there is no correct or incorrect answer, and where the choice depends entirely on the subjective value assigned to the different options? For example, if offered a choice between an apple and an orange, there is no correct or incorrect answer, the choice depends only on subjective preference and the respondent’s level of hunger.
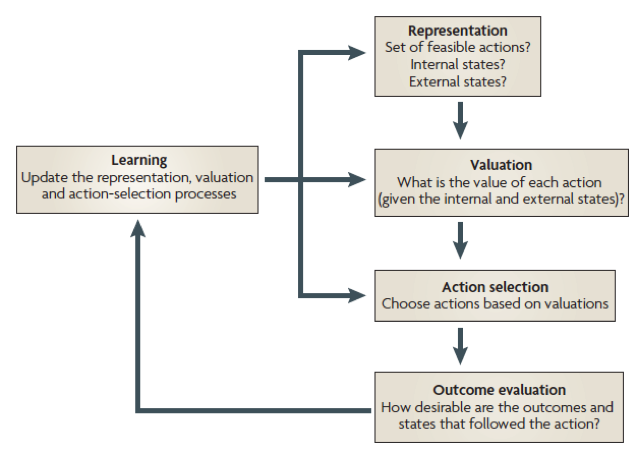
Figure 1. According to Rangel et al. (2008), value-based decision-making can be decomposed into five basic processes: representation of the problem, valuation of the different actions under consideration, selection of one action on the basis of the valuations, understanding the desirability of the outcomes that follow, and finally updating the decision-making processes to improve the quality of future decisions.
A classical framework developed by Rangel et al. (see Figure 1) proposed that when presented with several options, the brain assigns subjective values to each of them, then compares them and selects the one with the highest subjective value. According to this model, value-based decision-making can be broken down into at least three stages (Doya, 2008; Rangel et al., 2008; Sugrue et al., 2005) (see Figure 1). The first stage involves identifying the current situation (or state), including the internal state (e.g., hunger), the external state (e.g., cold), and potential courses of actions (e.g., to purchase food). Second, a valuation system attributes a subjective value to each option under consideration, weighting available options in terms of reward and punishment, as well as cost and benefit. Third, the agent selects an action based on this valuation, choosing the option with the highest assigned subjective value. Finally, the selected action may be re-evaluated based on the outcome, allowing the person to update their decision-making processes based on what they learned. Although these processes may occur in parallel, this simplified framework helps us understand basic computations performed by the brain. This framework shows that the brain must perform multiple computations to make simple decisions. It also indicates that valuation is a key stage in the subsequent selection of a given option.
It is unclear whether there are separate valuation systems in the brain. However, a number of studies identify at least two systems: Pavlovian and instrumental conditioning. In Pavlovian (or classical) conditioning, subjects learn to predict outcomes without ever having the opportunity to act. In instrumental conditioning, animals learn to choose actions to obtain rewards and avoid punishments. Various strategies are possible, such as optimizing the average rate of acquisition of rewards minus punishments, or optimizing the expected sum of future rewards, where outcomes received in the far future are discounted compared with outcomes received immediately.
The concept of common neural currency in the brain
Our behavior is motivated by different types of rewards among which we frequently need to choose. Because there is no single sense organ transducing rewards of different types, our brain must integrate and compare them to choose the options with the highest subjective value. It has been proposed that the brain uses a ‘common reward currency’ that functions as a scale to evaluate diverse behavioral acts and sensory stimuli (Sugrue et al., 2005). The need for this common currency arises from the variety of choices we face in our daily lives. Should I go to a movie or to a restaurant tonight? To make a choice, our brain must compare the values associated with each option.
Based on the ‘common currency’ concept, there should be a common brain network coding for different types of goods. Many functional magnetic resonance imaging (fMRI) studies are consistent with this idea, since common brain structures are involved in reward processing, regardless of reward nature. For example, increased midbrain, ventral striatum and orbitofrontal activities have been observed with different types of rewards, such as monetary gain (Abler et al., 2006; Dreher et al., 2006; J. P. O’Doherty, 2004), pleasant taste (McClure et al., 2003; J. O’Doherty, 2003; J. P. O’Doherty et al., 2003), beautiful faces (Bray & O’Doherty, 2007; Winston et al., 2007) and pain relief (Seymour et al., 2004, 2005, 2007). All of these neuroimaging studies only investigated one reinforcer at a time and did not compare any two reinforcers directly. Subsequent studies using fMRI in humans and electrophysiology in monkeys have identified the common and distinct brain networks involved in choosing between different options (Sescousse and Dreher, 2010; Sescousse et al., 2013). Together, the studies reviewed above indicate that the human ventromedial prefrontal cortex together with the ventral striatum is involved in encoding subjective value signals, consistent with the common currency hypothesis.
Delay discounting: neurodevelopmental studies and implications for education
A decision to engage in a given action is guided both by the prospect of reward and by the likely costs that this action entails. Psychological and economic studies have shown that outcome values are reduced when we are obliged to wait for them, an effect known as delay discounting. A classic experiment developed by Walter Mischel at Stanford University is known as the marshmallow experiment [1]. In this experiment, a child had to choose between one small but immediate reward, or two small rewards if they waited for a longer period of time. The reward was either a marshmallow or pretzel stick, depending on the child’s preference. The ability to wait a period of time to receive more rewards indicates greater patience and cognitive control. Cognitive control refers to the ability to override our impulses and make decisions based on goals rather than habits. Numerous studies have pointed out the crucial role of cognitive control in academic achievement (Duckworth et al., 2019). Individual differences in cognitive control reliably predict academic attainment and performance in standardized achievement tests. Of course, cognitive control is not a unique indicator of academic achievement. Other important factors, including socioeconomic status, general intelligence, motivation, and study skills contribute to academic achievement. However, cognitive control, and in particular the ability to resist immediate rewards to wait for greater delayed rewards is a robust measure across a number of academic outcomes. For example, children who are able to wait longer for the preferred rewards tend to have better life outcomes, as measured, for example, by SAT scores, educational attainment, or body mass index (BMI) (Duckworth et al., 2010; Mischel et al., 1988). The same pattern holds for other tasks requiring inhibition of automatic responses, sustained attention and retention of instructions in working memory. This suggests that the same brain networks responsible for cognitive control, such as the dorsolateral prefrontal cortex, may be at play across various domains to inhibit impulsive behavior.
Many smaller daily choices by students reflect a focus on academic goals that they value in the long run (e.g., doing one’s homework in order to later study medicine), and non-academic goals that they find more gratifying in the moment (e.g., going outside with friends without doing one’s homework). One key question is whether choosing the delayed option (staying at home to do homework) really reflects higher cognitive control or simply individual preferences for studying. Similarly, does a child who chooses the delayed reward of two marshmallows have especially strong cognitive control or simply a stronger preference for marshmallows? Observation of behavior alone is insufficient to answer this question but neuroimaging studies from the field of neuroeconomics may help.
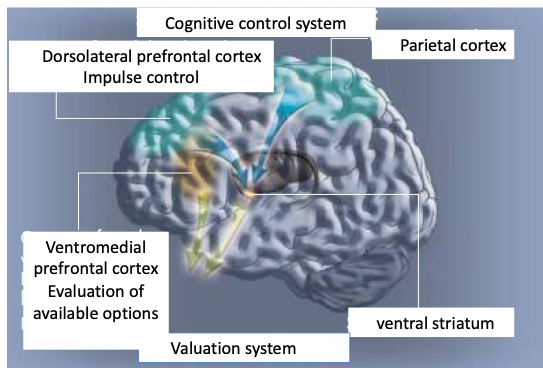
Figure 2. Scheme showing that the vmPFC/ventral striatum implements a valuation system under the cognitive control of the dorsolateral prefrontal cortex and parietal cortex, which inhibits impulsive behavior.
Early fMRI findings on delay-discounting support the finding that there are two separate systems in the brain: a limbic system that computes the value of rewards delivered immediately or in the near future based on a small discount factor, and a cortical system that computes the value of distant rewards based on a high discount factor (McClure et al., 2003; Schweighofer et al., 2007, 2008; Tanaka et al., 2004). Discounting results from the interaction of these two systems associated with different value signals. More recent studies indicate that there is a single valuation system discounting all future rewards (Kable & Glimcher, 2007). In this study, adult participants were asked to make choices between a small amount of money available now and a larger amount of money available in a few days or weeks. One problem with this type of study is that the choices are quite abstract and hypothetical since the rewards are not delivered while the participant is inside the scanner, nor do participants spend a long period inside the scanner. To remedy this, we have developed similar delayed discounting paradigms involving primary rewards (erotic stimuli) and real delay to experience inside the scanner (Prévost et al., 2008). These fMRI findings revealed that a similar ventromedial prefrontal cortex-ventral striatum brain network was engaged for delayed discounting of primary rewards. These results are consistent with the common currency hypothesis, suggesting that there is a single valuation system that discounts future rewards (Kable & Glimcher, 2007). These results, showing that the valuation system (vmPFC/ventral striatum) responsible for delay discounting co-exists with a parallel brain system responsible for cognitive control (e.g., Dorsolateral prefrontal cortex-intra parietal cortex) to inhibit impulsive behavior: the fronto-parietal network (see Figure 2). An important question that remains is how these two brain systems (cognitive control and valuation) communicate and interact when there is a need to inhibit impulsive or habitual behavior.
Adolescents and impulsivity: insights from studies of delay discounting
Our understanding of the brain mechanisms underpinning delay discounting in adults have important practical implications for adolescent impulsivity. Many behavioral studies have found higher impulsivity in adolescence. Early neuroscience studies have suggested that adolescent impulsivity may be attributed to the immaturity of the prefrontal cortex, which is necessary to exert cognitive control over urges originating in the limbic system. Changes occur over the adolescent decade in both grey and white matter volumes in prefrontal regions, suggesting that synaptic pruning and myelination enable more efficient and more effective self-regulation.
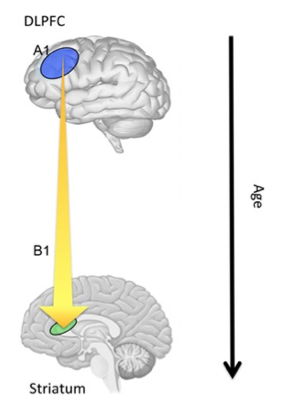
Figure 3. Compared to adults, adolescents are more likely to opt for smaller rewards sooner over larger ones later. As people get older, there is increased communication between the dorsolateral pre-fontal cortex (dlPFC) and the striatum, and as a result adults are less impulsive. The increased impatience of adolescents may thus be driven by weak cognitive control relative to adults (Bos et al., 2015).
More recent studies suggest that adolescent impulsivity is not simply due to immaturity of the prefrontal cortex, which is instrumental for cognitive control, but is accompanied by a temporary intensification of urges to pursue novel and rewarding experiences . The “maturational imbalance” view of adolescent impulsivity has long guided the study of adolescent risk-taking behavior. According to this view, adolescents’ disposition toward risk is because of a maturational imbalance between a brain network involved in cognitive control and goal-directed behavior and one involved in affective processes, including the anticipation and valuation of rewards. There is a rapid development of the reward system shortly after puberty, which produces increased sensitivity to reward. This declines in late adolescence. In contrast, structures of the cognitive control brain network that inhibit impulses and direct motivation toward goal-relevant behaviors continue to develop into a person’s thirties. However, the question remains whether the tendency of teenagers to choose immediate rewards is due to a lack of cognitive control regulating desires or to a higher sensitivity to rewards, which is also more prominent during adolescence.
A recent fMRI study in adolescents shed light on this question (Bos et al., 2015). It used a delayed discounting experiment in which teenagers had to choose between a small monetary reward given sooner and a greater one given later. Adolescents were more likely than adults to opt for the smaller rewards sooner (see Figure 3). This tendency to discount the future may be due to greater cognitive control, higher reward sensitivity, or both. Intertemporal preferences correlated with self-reported future orientation but not with hedonism. The choice of delayed reward over immediate reward was associated with increased engagement of the frontoparietal cognitive control network. Significantly, improvements in fronto-striatal connectivity with age coincided with increased preference for delayed rewards. As people get older there is increased communication between the dorsolateral pre-fontal cortex (dlPFC) and the striatum regions of the brain; thus, older participants, who have stronger connectivity between the dlPFC and the striatum were found to be less impulsive. Increased impatience among adolescents’ may be driven by weak cognitive control relative to adults, rather than by heightened reward sensitivity. This does not mean that impatient behavior is not the by-product of poor cognitive control and high reward sensitivity, but that there may be an imbalance between the two brain systems (cognitive control versus valuation) during adolescence.
Improving cognitive control functions through working memory training
Given the importance of self-control for academic achievement, intervention programs aiming to improve self-control in students are greatly needed. Identification of the brain changes occurring during such intervention programs is also crucial. One way to understand communication between the cognitive control brain system and the valuation brain system comes from recent findings related to working memory (WM), the capacity to maintain and manipulate information over a short period of time. When seeking to represent a delayed reward (e.g., studying to become a doctor or waiting to receive two marshmallows instead of one), working memory is needed. Some findings have shown that people with higher working memory capacity are also more patient and make less impulsive choices in delay discounting tasks. Perhaps more importantly, working memory performance can be trained through intervention programs. In a series of studies, Christos Constantinidis and Torkel Klingberg showed that working memory training for 14 hours per week over 5 weeks increases dlPFC activity, which is associated with cognitive control and reduced impulsivity. Working memory training not only increased the activity of neurons in the prefrontal cortex, it also increased the strength of connectivity within prefrontal cortex regions and between the prefrontal cortex and other areas. Neural changes after training were found in cortical areas that process spatial information in working memory as well as attention, potentially providing a basis for transfer to other cognitive and behavioral tasks that rely on spatial WM and spatially selective attention.
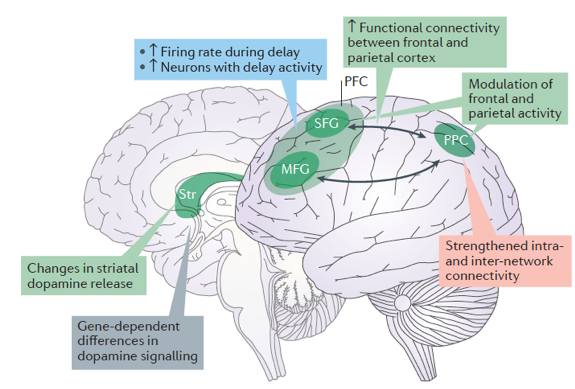
Figure 4. Summary of factors underlying training-induced increases in capacity. Training of working memory (WM) leads to a larger number of prefrontal cortex (PFC) neurons with delay activity and higher firing frequency during the delay period, and to stronger fronto-parietal functional connectivity with higher WM capacity and WM training. (Copyright Constantinidis 2016).
Therefore, WM training was found to increase plasticity of the cognitive control brain network. When we find ourselves in an environment that requires working memory, the cognitive control brain system becomes more plastic and is able to modulate the valuation system in order to be more patient. The brain becomes less sensitive to immediate rewards and more tolerant to frustration and delay. In addition to this neuroplasticity at the system level, researchers also observed changes in functional connectivity that occur at rest between frontal and parietal regions associated with WM training (Constantinidis & Klingberg, 2016; Jolles et al., 2013; Thompson et al., 2016). These changes in connectivity can be also observed with techniques other than fMRI, such as transcranial magnetic stimulation (TMS) applied over the parietal cortex propagates over the cortex, as measured by electroencephalography (EEG). Following WM training, TMS increased signals in the frontal and temporal lobe, demonstrating that training led to an increase in functional connectivity during task performance. The mechanisms underlying changes in functional connectivity may provide stronger synaptic connectivity between neurons or an activity-dependent increase in the myelination of the connecting axons (Gibson et al., 2014; Yeung et al., 2014). Consistent with this latter finding, WM capacity is associated with white-matter volume and structure and specifically with increased white-matter density in the parietal lobe after WM training (Takeuchi et al., 2010).
What happens when working memory capacity is reduced rather than trained? This can easily be manipulated by holding a distracting series of numbers in working memory. In this case, people became more inclined to choose immediate over delayed rewards. These results confirm that ‘free’ working memory capacity is needed for efficient cognitive control. In addition, children performing a delayed discounting experiment with progressively increasing delays tend to choose the delayed option more frequently than the immediate reward option. This type of training has also been shown to modify the brain system engaged in cognitive control.
Conclusions
In recent years, neuroeconomics has helped to extend the study of value-based decision-making (e.g., delay discounting, risky decision-making, and loss aversion) beyond the healthy adult population, including adolescents, older adults and even children. Neurodevelopmental studies help us understand the emergence and neural underpinnings of increased impulsivity and risk-seeking in adolescents. Intervention programs such as working memory training yield evidence that the brain systems underlying this function are plastic. Improvements in working memory have been reported for a variety of populations including typically developing pre-school- and primary-school-aged children, children with Attention Deficit Hyperactivity Disorder (ADHD) who display elevated levels of hyperactive and inattentive behavior, and in children with poor working memory. These findings obtained from recent studies have begun to be successfully applied by teachers in the school environment (Holmes & Gathercole, 2014).
References
Abler, B., Walter, H., Erk, S., Kammerer, H., & Spitzer, M. (2006). Prediction error as a linear function of reward probability is coded in human nucleus accumbens. Neuroimage, 31, 790–795.
Bos, W. van den, Rodriguez, C. A., Schweitzer, J. B., & McClure, S. M. (2015). Adolescent impatience decreases with increased frontostriatal connectivity. Proceedings of the National Academy of Sciences, 112(29), E3765–E3774. https://doi.org/10.1073/pnas.1423095112
Bray, S., & O’Doherty, J. (2007). Neural coding of reward-prediction error signals during classical conditioning with attractive faces. J Neurophysiol, 97, 3036–3045.
Constantinidis, C., & Klingberg, T. (2016). The neuroscience of working memory capacity and training. Nature Reviews Neuroscience, 17(7), 438–449. https://doi.org/10.1038/nrn.2016.43
Doya, K. (2008). Modulators of decision-making. Nat Neurosci, 11, 410–416.
Dreher, J. C., Kohn, P., & Berman, K. F. (2006). Neural coding of distinct statistical properties of reward information in humans. Cereb Cortex, 16, 561–573.
Duckworth, A. L., Taxer, J. L., Eskreis-Winkler, L., Galla, B. M., & Gross, J. J. (2019). Self-Control and Academic Achievement. Annual Review of Psychology, 70(1), 373–399. https://doi.org/10.1146/annurev-psych-010418-103230
Duckworth, A. L., Tsukayama, E., & Geier, A. B. (2010). Self-controlled children stay leaner in the transition to adolescence. Appetite, 54(2), 304–308. https://doi.org/10.1016/j.appet.2009.11.016
Gibson, E. M., Purger, D., Mount, C. W., Goldstein, A. K., Lin, G. L., Wood, L. S., Inema, I., Miller, S. E., Bieri, G., Zuchero, J. B., Barres, B. A., Woo, P. J., Vogel, H., & Monje, M. (2014). Neuronal Activity Promotes Oligodendrogenesis and Adaptive Myelination in the Mammalian Brain. Science. https://doi.org/10.1126/science.1252304
Holmes, J., & Gathercole, S. E. (2014). Taking working memory training from the laboratory into schools. Educational Psychology, 34(4), 440–450. https://doi.org/10.1080/01443410.2013.797338
Jolles, D. D., van Buchem, M. A., Crone, E. A., & Rombouts, S. A. R. B. (2013). Functional brain connectivity at rest changes after working memory training. Human Brain Mapping, 34(2), 396–406. https://doi.org/10.1002/hbm.21444
Kable, J. W., & Glimcher, P. W. (2007). The neural correlates of subjective value during intertemporal choice. Nature Neuroscience, 10(12), 1625–1633. https://doi.org/10.1038/nn2007
McClure, S. M., Berns, G. S., & Montague, P. R. (2003). Temporal prediction errors in a passive learning task activate human striatum. Neuron, 38, 339–346.
Mischel, W., Shoda, Y., & Peake, P. K. (1988). The nature of adolescent competencies predicted by preschool delay of gratification. Journal of Personality and Social Psychology, 54(4), 687–696. https://doi.org/10.1037/0022-3514.54.4.687
O’Doherty, J. (2003). Can’t learn without you: Predictive value coding in orbitofrontal cortex requires the basolateral amygdala. Neuron, 39, 731–733.
O’Doherty, J. P. (2004). Reward representations and reward-related learning in the human brain: Insights from neuroimaging. Curr Opin Neurobiol, 14, 769–776.
O’Doherty, J. P., Dayan, P., Friston, K., Critchley, H., & Dolan, R. J. (2003). Temporal difference models and reward-related learning in the human brain. Neuron, 38, 329–337.
Prévost, C., Pessiglione, M., Cléry-Melin, M.-C., & Dreher, J.-C. (2008). Delay versus effort-discounting in the human brain. In Society for Neuroscience.
Rangel, A., Camerer, C., & Montague, P. R. (2008). A framework for studying the neurobiology of value-based decision-making. Nature Reviews. Neuroscience, 9(7), 545–556. https://doi.org/10.1038/nrn2357
Schweighofer, N., Bertin, M., Shishida, K., Okamoto, Y., Tanaka, S. C., Yamawaki, S., & Doya, K. (2008). Low-serotonin levels increase delayed reward discounting in humans. J Neurosci, 28, 4528–4532.
Schweighofer, N., Tanaka, S. C., & Doya, K. (2007). Serotonin and the evaluation of future rewards: Theory, experiments, and possible neural mechanisms. Ann N Y Acad Sci, 1104, 289–300.
Seymour, B., O’Doherty, J. P., Dayan, P., Koltzenburg, M., Jones, A. K., Dolan, R. J., Friston, K. J., & Frackowiak, R. S. (2004). Temporal difference models describe higher-order learning in humans. Nature, 429, 664–667.
Seymour, B., O’Doherty, J. P., Koltzenburg, M., Wiech, K., Frackowiak, R., Friston, K., & Dolan, R. (2005). Opponent appetitive-aversive neural processes underlie predictive learning of pain relief. Nat Neurosci, 8, 1234–1240.
Seymour, B., Singer, T., & Dolan, R. (2007). The neurobiology of punishment. Nat Rev Neurosci, 8, 300–311.
Sugrue, L. P., Corrado, G. S., & Newsome, W. T. (2005). Choosing the greater of two goods: Neural currencies for valuation and decision-making. Nat Rev Neurosci, 6, 363–375.
Takeuchi, H., Sekiguchi, A., Taki, Y., Yokoyama, S., Yomogida, Y., Komuro, N., Yamanouchi, T., Suzuki, S., & Kawashima, R. (2010). Training of Working Memory Impacts Structural Connectivity. Journal of Neuroscience, 30(9), 3297–3303. https://doi.org/10.1523/JNEUROSCI.4611-09.2010
Tanaka, S. C., Doya, K., Okada, G., Ueda, K., Okamoto, Y., & Yamawaki, S. (2004). Prediction of immediate and future rewards differentially recruits cortico-basal ganglia loops. Nat Neurosci, 7, 887–893.
Thompson, T. W., Waskom, M. L., & Gabrieli, J. D. E. (2016). Intensive Working Memory Training Produces Functional Changes in Large-scale Frontoparietal Networks. Journal of Cognitive Neuroscience, 28(4), 575–588. https://doi.org/10.1162/jocn_a_00916
Winston, J. S., O’Doherty, J., Kilner, J. M., Perrett, D. I., & Dolan, R. J. (2007). Brain systems for assessing facial attractiveness. Neuropsychologia, 45, 195–206.
Yeung, M. S. Y., Zdunek, S., Bergmann, O., Bernard, S., Salehpour, M., Alkass, K., Perl, S., Tisdale, J., Possnert, G., Brundin, L., Druid, H., & Frisén, J. (2014). Dynamics of Oligodendrocyte Generation and Myelination in the Human Brain. Cell, 159(4), 766–774. https://doi.org/10.1016/j.cell.2014.10.011