The neurodevelopmental underpinnings of children’s learning: Connectivity is key
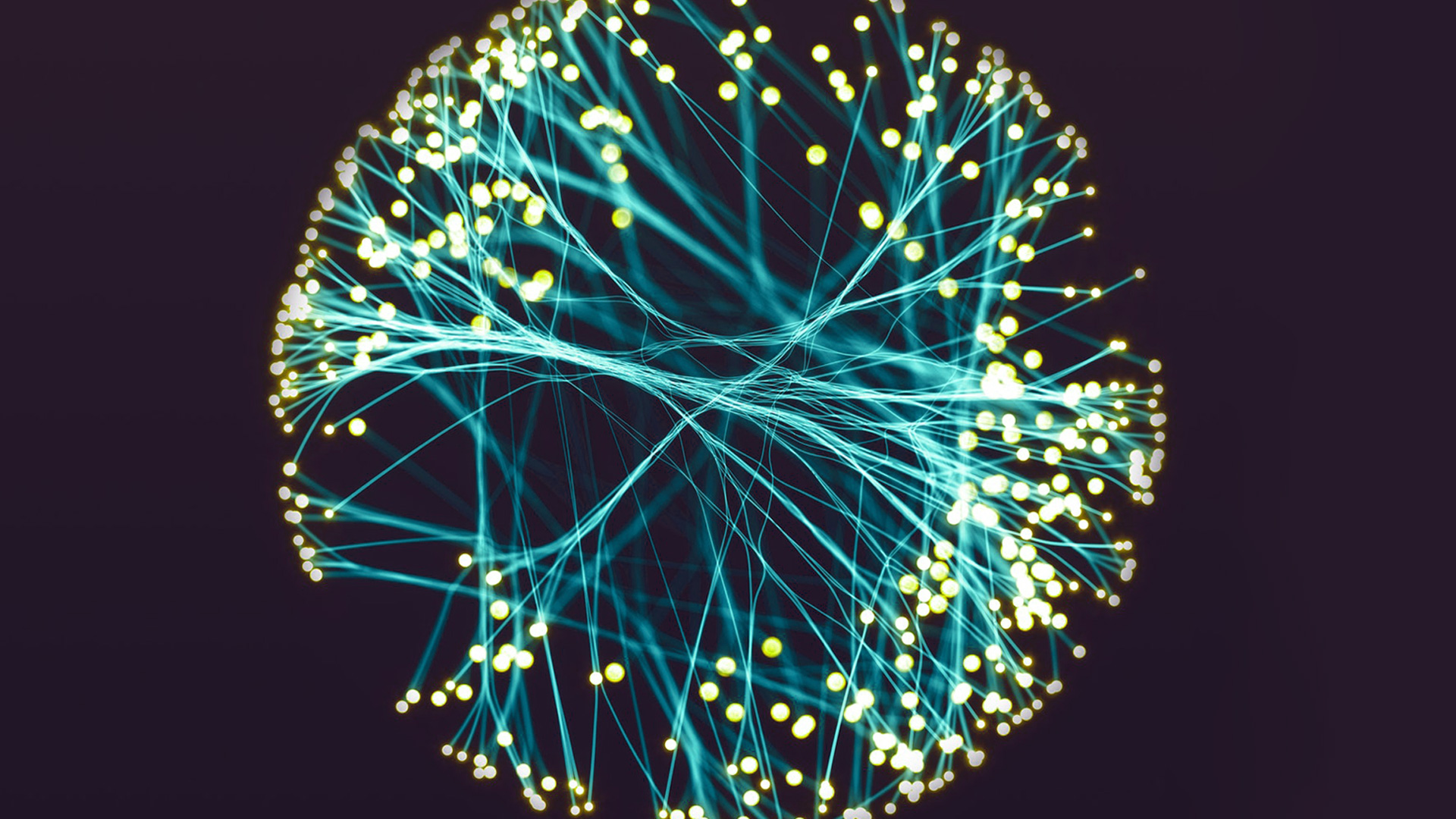
Executive summary.
- Continuous adaptation of the functional and structural connections within and between neural networks provides a primary mechanism of neuroplasticity within the brain.
- Connectivity provides the basis for both short- and long-range inter-cellular communication.
- Connectivity is a lifelong neurodevelopmental process and provides a key neurophysiological mechanism of learning.
- Variability from the typical patterns of brain connectivity through development and resulting from learning, provide new windows into understanding learning disabilities.
Brain connectivity is a primary mechanism of neuroplasticity
Neurons are the basic functional unit of the brain, providing the electro-chemical signalling that serves as the main currency of information. Individual neurons can be thought of as rather simple biological batteries, each maintaining a gradient of biochemical ions across its cell membrane, which results in a small, local electrical charge – or potential (Figure 1, left panel). The right panel of Figure 1 shows the main components of a prototypical neuron. Incoming signals from neighbouring brain cells are communicated to the neuron’s dendrites and act to continuously modify the magnitude of the neuron’s electrical charge. When the sum of signals from other neurons drives the electrical gradient to and then past a critical voltage, an electrical signal – the action potential – is generated and propagated along the neuron’s axon. This signal ultimately modulates the activity of other neurons to which it connects. This process of synaptic transmission comprises the release of neurotransmitter chemicals at the junction between two cells – the synapse. Neurotransmitters released in response to the action potential in the pre-synaptic cell bind to receptors on the dendrites of the post-synaptic cell. The effects of such neurotransmitter binding serve to modify the electrical potential in the cell, either exciting it toward generating an action potential or inhibiting it from doing so.
Of course, this electro-chemical process for deriving and propagating neural signals in individual cells within the brain actually takes place on a massive scale. An individual neuron, for which there are upwards of approximately 85 billion in a single human brain1, may interact through synapses with up to 15,000 others, providing the basis of networks for neuronal communication on an enormous scale. Neurons rarely function in isolation, therefore. Instead, they adapt both their structure and function in a near continuous fashion in response to both their local environment within the brain and to the outside world. This neuroplasticity acts to maximise the efficiency and fidelity with which neural networks can respond to and transmit information. Cognition – our thinking, reasoning and learning processes – are derived from activity in neural networks within the brain, on a spatial scale that is intermediate between that which is highly localised within discrete and specific brain regions and that which is highly diffuse and distributed across the entire volume of the brain2. These spatial properties, through which a balance between local and more global processing of information is achieved, provide an organisational hierarchy that enables the component neural systems that underlie our ability to think, reason, and to choose relevant behavioural responses, to be both functionally integrated and differentiated.
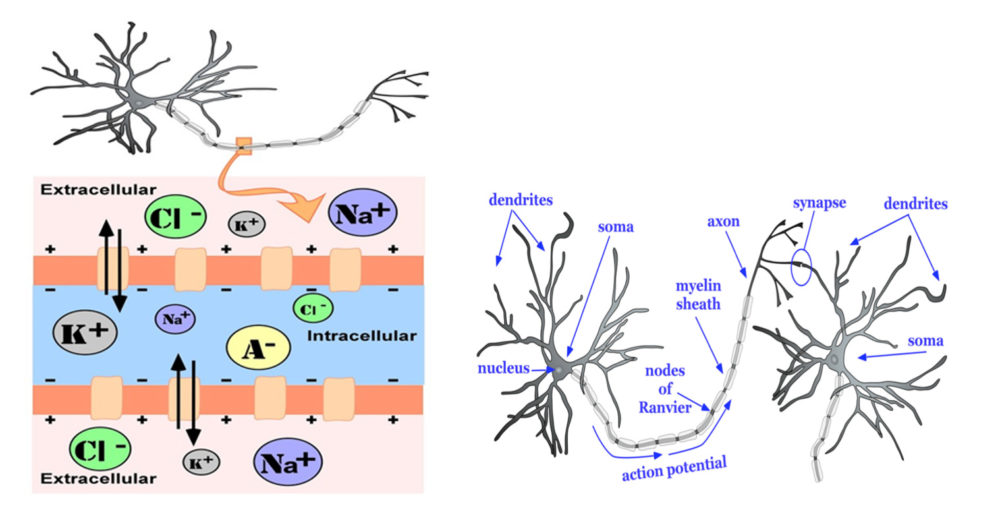
Figure 1. Left panel. Cross section of a prototypical membrane of a neuron. The intracellular environment with high potassium (K+) and organic anions (A-) is actively maintained by a sodium/potassium (NA+) pump (bidirectional arrows) which facilitates ion exchange across the cell membrane. This ion exchange results in a small negative charge that is maintained across the membrane as its default (resting) state. Right panel. A simple neural network comprising two prototypical neurons, highlighting four main structural features that characterise all nerve cells: a cell body, or soma; an axon terminating at synapses between the pre-synaptic (left) and post-synaptic (right) cells, and a set of dendrites for receiving incoming signals. Illustrations from reference [3] and made available through Creative Commons license (CC by 4.0).
Neurodevelopment: Implementing neural connectivity
Neurodevelopment is a lifelong process involving the modification of the structural and functional properties of the brain, driven by both genetic and environmental influences and their interaction. Figure 2 shows the sequence of critical neurodevelopmental stages across the lifespan, with links to observed patterns of data obtained with non-invasive neuroimaging paradigms used for the measurement and visualisation of brain structure and function.
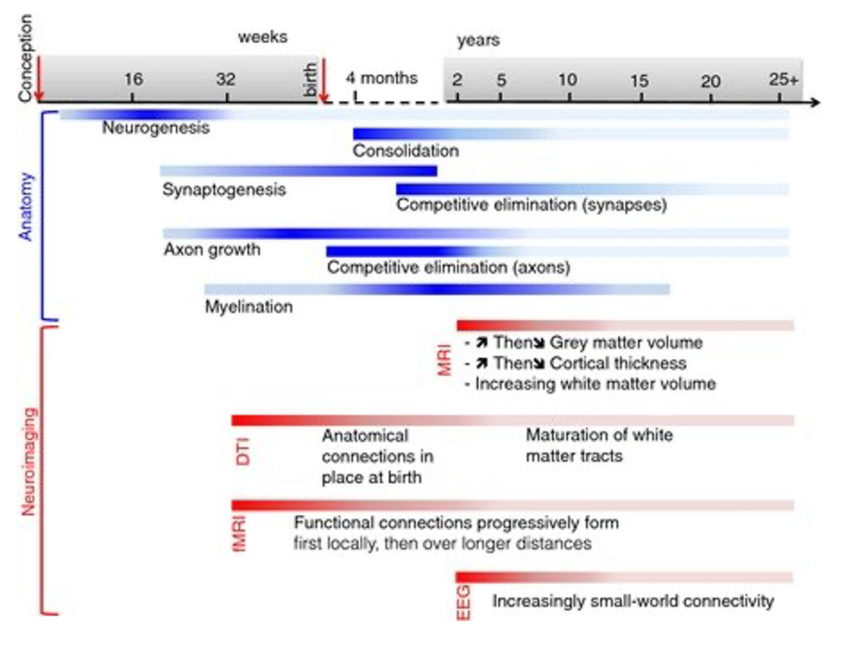
Figure 2. The sequence of key neurodevelopmental events in the maturation of human brain structure (blue bars). Each bar represents the typical timeframe over which a particular process is active, with darker shading marking time periods of peak changes. Red bars indicate anatomical observations of these phenomena, as visualised with different neuroimaging paradigms: fMRI, functional Magnetic Resonance imaging; DTI: Diffusion tensor imaging; EEG: electroencephalography. The mechanisms that directly underlie the establishment of neural connectivity in the developing brain are particularly active in the early to mid-childhood and parallel the particularly steep gains in learning potential observed for children during this time period. Image from reference [4] available by permission through Creative Commons license (CC BY 3.0).
The development of connectivity for learning: ‘What fires together, wires together’
Structural and functional connectivity provides mechanisms for implementing adaptation of the brain in response to an individual’s experience of the world. As children are born with nearly a full complement of brain cells, adaptation of responses to environmental change- the underlying basis of learning for any organism- is accomplished mainly through modifying neural connectivity. Connectivity increases in parallel with children’s advancement of their cognitive capacities and learning achievement5 (Figure 2, blue bars & Figure 3).
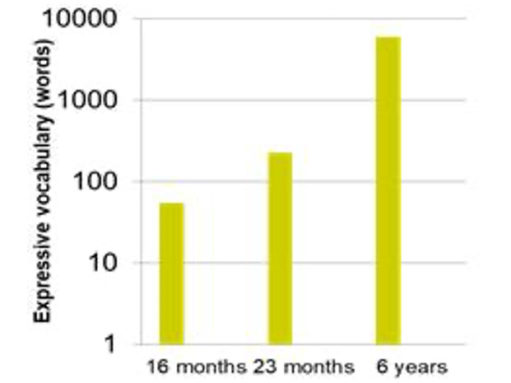
Figure 3. Estimates of the gains in expressive vocabulary in young childhood (see for e.g., refs [6,7]). The rapid development in children’s vocabulary learning in early childhood parallels the changes in functional connectivity in the brain.
Describing connectivity in the brain
The scale of connectivity within the brain is of a scale so large that neuroscientists have adapted interdisciplinary methods first developed in mathematics and the physical sciences to describe the spatial relationships (topologies) involved11. One promising method for describing connectivity patterns in the brain – the ‘connectome’ – is to employ techniques previously used for describing the efficiency of information processing in non-biological networks12. These models describe connectivity patterns in networks of spatially distributed units (e.g., individuals or neurons), as shown in Figure 4.
By analogy, consider a graphical depiction of the relationships between family members (neurons), both to each other and to increasingly more distant individuals (or groups) at various spatial scales in the outside world (e.g., houses, streets, cities, states, countries, world hemispheres). A single individual and the persons with whom they live in close spatial proximity can be seen to comprise a densely connected local network or processing hub. Information processing efficiency within the hub is maximised by establishing high interconnectivity between individual members. Members within individual hubs also establish connections with other individuals, located in other hubs, at varying distances from their home network. These networks can exist independently in the local community or more distally to the home network, with the efficiency of communication between them achieved through a few robust connections with these more distant groups. Overall processing in the system (the brain) can be summarised in terms of its overall processing efficiency, described in terms of both the local clustering and the typical length of the paths between hubs.
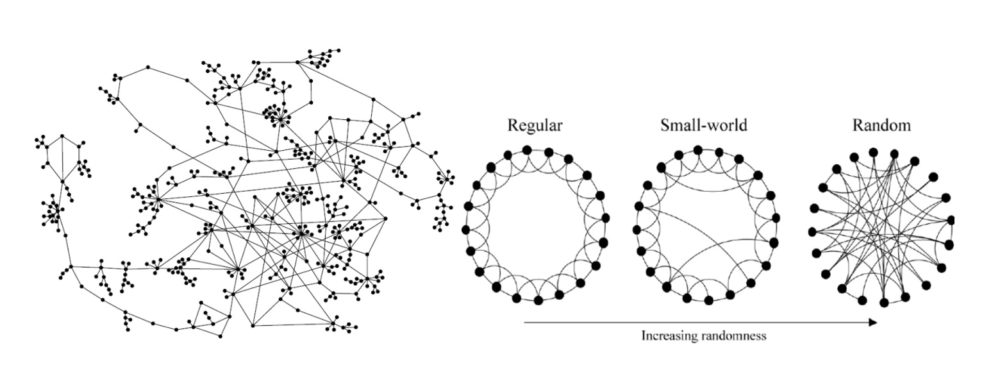
Figure 4. Schematic diagrams of connectivity patterns in networks. Left panel: Individual units (in this case people, not neurons) are shown as black dots, with their inter-relationships depicted by the lines connecting them. The overall network topology can be described in terms of the clustering of individuals in a local level and by the average length of the path between individuals distributed at distance more globally (Figure from reference [13]). Right panel. Graphs of hypothetical networks, described connectivity on both local and more global scales. Regular networks can be represented by a pattern of systematic interconnections between local neighbours, but in the comparative absence of longer-range connections, resulting in high local processing efficiency, but lower global processing efficiency. Random networks are represented by their unpredictable connections between units distributed both locally and more globally, resulting in higher global processing efficiency but lower local processing efficiency compared to a regular network. A Small World network represents an optimised connectivity pattern for the system as a whole, achieving both high local and high global processing efficiency, by having a short average length of a path between hubs in the network, along with high local clustering of functional units. (Figure from reference [11])
As applied to descriptions of developing brain, a particular strength of these schematic approaches to network connectivity is that they may provide particularly parsimonious descriptions of how connectivity varies across children with different developmental trajectories. Figure 5 shows two hypothetical developmental trajectories that may be particularly relevant for understanding the mechanisms by which children’s neurocognitive development varies across individuals.
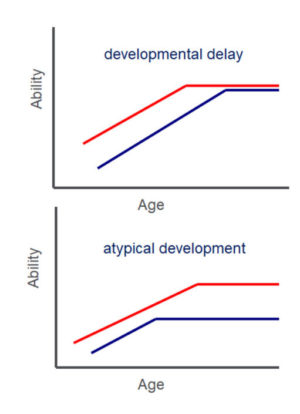
Figure 5. Hypothetical developmental trajectories, describing changes in an outcome measure of interest (labelled here’ ability’) and across age. Red lines represent the normative developmental trajectory of ability; blue lines represent the trajectory of a single individual. The developmental delay trajectory is characterised by a rightward shift in the individual measure of ability towards later ages, the result being that normative ability is achieved by the individual but at an age later than is typical for the normative group. The atypical development trajectory represents a pattern of ability in which normative performance is not attained by the individual at any point (age) across development.
Using graph theory, individual connectivity data obtained with appropriate neuroimaging techniques for measuring brain structure or function (see for e.g., ref [4]), can be described either longitudinally or at a single timepoint, and compared across groups or other individuals, to evaluate the extent to which there is a deviation from the normative developmental trajectory. For example, a child with a developmental delay might be described as having functional brain connectivity observed as a typical progression towards a Small World network (see top of Figure 4), similar to that of a younger, typically developing child. In contrast, a developmental trajectory that is atypical and which might not be predicted to resolve through maturation or normal environmental exposure (e.g., normal classroom instruction within an educational environment) might be described in terms of brain connectivity patterns that do not match the typical developmental trajectory for children at any given age (for e.g., a more randomly connected network as shown in the bottom of Figure 4). This information may be particularly informative in aiding the understanding of the different mechanisms of underachievement in learning by children. Children with similar patterns of low scores on behavioural measures (of educational achievement, for example) may arrive at this profile through different developmental mechanisms. Appreciating that potentially different trajectories may characterise the neurodevelopmental patterns of individuals could powerfully impact the choice of intervention strategy that is applied. For example, a trajectory of simple developmental delay might be predicted to be remediated through developmental maturity and via regular exposure to relevant environmental stimuli. In contrast, a trajectory identified as atypical might warrant more require more intensive and individually tailored support to remediate the difficulties encountered.
Neurodevelopmental disorders of learning as disorders of connectivity
Unexplained learning difficulties are common in the world’s population. Between 14% and 30% of school-aged children require additional support for their learning14,15. In the developing brain, functional and structural connectivity underpins the refinement of cognitive ability and achievement. Cognitive behaviour, and particularly more complex learned skills that are unique to humans, such as reading and mathematics, are implemented through distributed networks of brain areas that depend upon the fine-grained and temporally precise coordination of information between their processing hubs (see Figure 6). This is coupled with the necessity for the network to adapt and modify its activity in response to the repeated presentation of associations between relevant and meaningful environment stimuli.
There is a strong evidence base for structural and functional differences between the brains of persons with different types of learning difficulties, compared to typically-developing individuals16-19. The emphasis of most of these previous studies has been on identifying particular regions of interest in the brain that contribute to the cognitive ability under investigation, and toward identifying the extent to which measures of neural activity in these areas differ between groups of persons either with or without specific types of deficits measured behaviorally. In functional studies neuroimaging tasks that simulate different learning domains (e.g., reading, math, language processing) are typically used to identify the brain components that are under- or over-active in response to the processing demands of the task. An example image of this type of study is shown in Figure 6, for word reading during the acquisition of a second language, and identifies key areas for which cellular activity is driven by particular reading component skills.
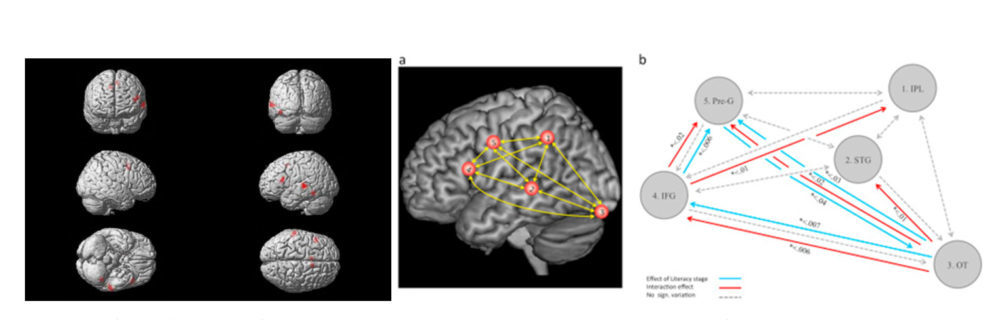
Figure 6. Left panel. Shows an fMRI image obtained during a reading task. Brain responses for letter strings and words were compared during the process of learning an additional language. Coloured areas show that reading particularly engages a set of (predominantly) left-hemisphere lateralised areas in the inferior frontal gyrus, temporo-parietal cortex and inferior temporal cortex which are also functionally linked to the component reading skills of semantic, phonological and orthographic processing. Middle and right panels (a, b). Describing functional connectivity within key hubs of the reading network (a. areas labelled 2,3,4 correspond with those identified in the left panel) including, b. a graph of the changes in functional connectivity during reading skill acquisition and their directional effects associated with different trajectories of reading acquisition (coloured arrows). Note here the change of emphasis from that of localised activity (left panel) to describing functional connectivity patterns between hubs in a network of brain areas engaged in a task (right panels). Images from reference [21] are available by permission through CreativeCommons license (CC BY-NC-ND 4.0)
Neuroimaging studies of learning, both for typical and atypical development, are being extended beyond more modular accounts of region-specific information processing, to focus more on how information is represented and processed within the more distributed brain networks that underlie the individual differences in cognition2. For example, recent investigations have described the normative patterns in the functional connection strengths between hubs in the reading network, their differences between readers with atypical and typical reading development, and how their connectivity changes with learned experience and skill acquisition (e.g., right panels in Figure 6). Patterns of experience-dependent connectivity in the brain differ between individuals and are associated with their variable profiles of learning achievement20,21. These relationships are, of course, bidirectional: existing structural and functional differences that precede the onset of initial or continued skill learning constrain to some degree ultimate learning outcomes, but brain structure and function can also change dramatically in response to environmental factors including learning environments. Differences in connectivity patterns will therefore ultimately find utility for describing not only group differences at a single timepoint, but also how individual developmental trajectories characterise changes in learning and learning potential over time.
Summary
Learning in the brain is implemented through the modification of structural and functional connectivity between aggregates of neurons or networks. Network organisation underpins the efficiency with which information is communicated, with higher processing efficiency achieved by connectivity patterns that are locally dense but much sparser when the distance between local hubs becomes longer. Neurodevelopment can be described in terms of the changes that approximate these maximally efficient Small World networks, including trajectories associated both with typical and atypical development. These different developmental trajectories can be used to understand differences in the structural-functional relationships of the brain that are observed in some of the most commonly diagnosed disorders of childhood that manifest as differences in children’s learning outcomes.
References
- von Bartheld, C.S., Bahney, J., & Herculano-Houzel, S. (2016). The search for true numbers of neurons and glial cells in the human brain: A review of 150 years of cell counting. The Journal of Comparative Neurology, 524(18), 3865–3895. https://doi.org/10.1002/cne.24040
- Rabinovich, M.I., Simmons, A.N., & Varona, P. (2015). Dynamical bridge between brain and mind. Trends in Cognitive Sciences,19(8), 453–461. https://doi.org/10.1016/j.tics.2015.06.005
- Furtak, S. (2020). Neurons. In R. Biswas-Diener & E. Diener (Eds), Noba textbook series: Psychology. DEF publishers. http://noba.to/s678why4
- Vértes, P., & Bullmore, E. (2015). Annual research review: Growth connectomics – The organisation and reorganisation of brain networks during normal and abnormal development. Journal of Child Psychology & Psychiatry, 56(3), 299–320. https://doi.org/10.1111/jcpp.12365
- Sripada. C., Angstadt, M., Rutherford, S., et al. (2020) Brain connectivity patterns in children linked to neurocognitive abilities. bioRxiv. https://doi.org/10.1101/2020.09.10.291500
- Fenson, L., Dale, P., Reznick, J., et al. (1994). Variability in early communicative development. Monographs of the Society for Research in Child Development, 59(5), 1–185. https://doi.org/10.2307/1166093
- Munro, N., & McGregor, K. (2015). Semantics. In S. McLeod and J. McCormack (Eds.), Introduction to speech, language and literacy. (pp. 181–230). Oxford University Press.
- Hebb D.O. (1949). The organisation of behaviour. John Wiley and Sons.
- Fox, K., & Stryker, M. (2017). Integrating Hebbian and homeostatic plasticity: Introduction. Philosophical Transactions of the Royal Society of London, Series B, Biological Sciences, 372(1715). https://doi.org/10.1098/rstb.2016.0413
- Langille, J.J., & Brown, R.E. (2018). The synaptic theory of memory: A historical survey and reconciliation of recent opposition. Frontiers in Systems Neuroscience, 12. https://doi.org/10.3389/fnsys.2018.00052
- Watts, D.J., & Strogatz, S.H. (1998). Collective dynamics of ‘small-world’ networks. Nature, 393(6684), 440–442. https://doi.org/10.1038/30918
- Latora, V., & Marchiori, M. (2001). Efficient behavior of small-world networks. Physical Review Letters, 87, 198701. https://doi.org/10.1103/PhysRevLett.87.198701
- Potterat, J.J., Muth, S.Q., Rothenberg, R.B., et al. (2002). Sexual network structure as an indicator of epidemic phase. Sexually Transmitted Infections, 78, i152–i158.
- Department for Education. (2020). Special educational needs and disability: an analysis and summary of data sources. DfE-00072-2020.
- Drabble, S. (2013). Support for Children with Special Educational Needs (SEN). European Union. https://www.rand.org/pubs/research_reports/RR180.html.
- Pugh K.R., Mencl, W.E., Shaywitz B.A., et al. (2000). The angular gyrus in developmental dyslexia: Task-specific differences in functional connectivity within posterior cortex. Psychological Science, 11(1), 51–56. https://doi.org/10.1111/1467-9280.00214
- Aboud, K.S., Bailey, S.K., Petrill, S.A., & Cutting, L.E. (2016), Comprehending text versus reading words in young readers with varying reading ability: distinct patterns of functional connectivity from common processing hubs. Developmental Science, 19, 632–656. https://doi.org/10.1111/desc.12422
- Jolles, D., Ashkenazi, S., Kochalka, J., et al. (2016). Parietal hyper‐connectivity, aberrant brain organisation, and circuit‐based biomarkers in children with mathematical disabilities. Developmental Science, 19, 613–631. https://doi.org/10.1111/desc.12399
- Liu, L., Li, H., Zhang, M., Wang, Z., Wei, N., Liu, L., Meng, X., & Ding, G. (2016). Aberrant topologies and reconfiguration pattern of functional brain network in children with second language reading impairment. Developmental Science, 19, 657–672. https://doi.org/10.1111/desc.12440
- Siugzdaite, R., Bathelt, J., Holmes, J., & Astle, D.E. (2020). Transdiagnostic brain mapping in developmental disorders. Current Biology, 30, 1245–1257. https://doi.org/10.1016/j.cub.2020.01.078
- Morken, F., Helland, T., Hugdahl, K., & Specht, K. (2017). Reading in dyslexia across literacy development: A longitudinal study of effective connectivity. NeuroImage, 144, 92–100. https://doi.org/10.1016/j.neuroimage.2016.09.060