Wearable technology for neuroscience in classrooms
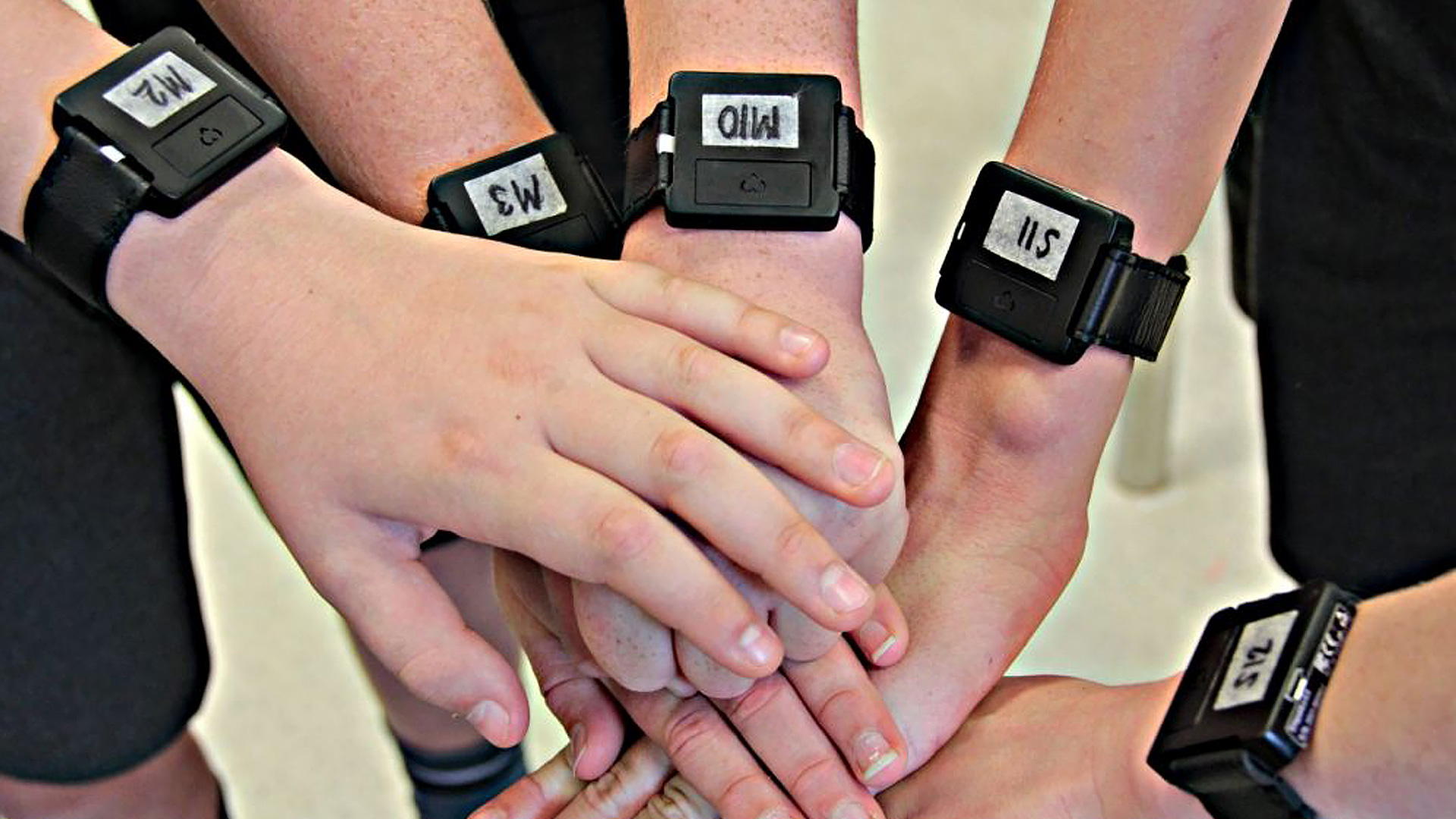
Executive Summary
- Sociometric Badges can be used to quantify interpersonal interactions, of who is interacting with whom, how long they spend talking, and how long they spend listening1.
- Biometric wristbands can provide measures of relative changes in students’ physiological arousal levels, representing cognitive engagement or stress responses to events in the classroom2.
- Wireless EEG brain headsets are the least developed technology for education research but they can provide direct measurements of the brain states of the learners during learning activities3.
- The development of computational techniques are just as crucial as the hardware development of the various sensors in ascertaining the engagement and connectedness in the classroom.
- One of the main challenges is to provide simple but powerful metrics that are useful and meaningful to educators in relation to student engagement or pedagogical practice.
Introduction
Education neuroscience is a new and rapidly developing field that aims to link psychology and neuroscience research on how the brain learns with education research on teaching and learning. Typically, cognitive and brain research is conducted in the laboratory under tightly controlled experimental conditions, and presents particular problems in the dynamic and sometimes unpredictable environment of school classrooms.
There is currently a lot of interest in wireless, wearable technology devices that allow relatively naturalistic measurement of students’ social, biological, or physiological states during regular learning activities in the classroom.
Wearable technology devices are easily removable items such as watches, jewelry, caps or headbands, fabrics and textiles that can measure data on the wearer’s activity level, social interactions, or physiological states. Such devices usually provide information back to the wearer through the device, via mobile phone or tablet applications, or via cloud internet services. There has been an incredible growth in the general use of wearable devices such as activity and fitness bands, and the global market for wearable technology is expected to reach over US$50 billion by 20224.
In education, these devices can provide automated and naturalistic measurement of data relating to students’ engagement, social interactions, emotional states, and even brain states during classroom learning activities. A particular challenge for this area is the development of computational methods that can translate the biometric data collected into metrics that are meaningful and useful for educators in relation to teaching and learning practices.
At present such wearable technology and the development of computational methods to deal with the data are at the forefront of research in education neuroscience. As research tools, wearable devices have enormous potential to bring further understanding of the influence of classroom and pedagogical practices on the physiological and brain states of students as learners. These methods also provide a bridge between education and the enormous body of knowledge that exists in psychology and neuroscience on brain processes for attention, cognition, memory, and learning.
But as computational methods and real-time metrics are developed, this opens possibilities for wearable technology to be used as teacher aids rather than only as research tools. In this area, there are still many social and ethical issues to address. Imagine a simple wristband that can provide the teacher with a real-time measure of “engagement level” for every student in the class via a mobile tablet. Or imagine a wireless headset that could detect real-time “confusion level” in students and feed back to an artificial intelligence digital learning computer program to alter the content being delivered to the student.
These technologies are certainly possible in the near future, and with the enormous global value of wearable technology, these devices are likely to be marketed very heavily towards schools and educational organisations. The challenge for educators is to consider how best these devices and analysis methods could be adapted for use in classrooms if they are to really benefit teaching and learning.
Below I review the main types of wearable technology for use in classrooms and issues around data analysis and use as research tools or as teacher aids.
Sociometric Badges
Sociometric badges provide measurement of social interactions between individuals. One popular device is a credit-card sized badge that can be worn on a lanyard around the neck, but the same sensors could easily be built into a headband or a wristband watch. They have been used for assessment of inter-personal communication and social interactions in business environments, around boardroom meeting tables and around office workplaces. They can also be used to quantify student-student and teacher-student social and verbal interactions in the classroom.
These badges can include sensors that detect proximity to other badges, including the duration of face-to-face interactions, and audio sensors for detecting speech interactions, including the time spent talking and the time spent listening.
The proximity sensors can detect which people are close together at any point in time. They often use Bluetooth signals and can detect the strength of the other badge signals to give an indication of who is nearby the wearer and how close they are at any time. As such wireless signals are non-directional, these sensors cannot detect whether people are in front of or behind the wearer and whether or not they are directly interacting with the wearer. Some badges also contain infrared sources and sensors that will detect face-to-face proximity to other badges. This can give an additional measure of the duration of face-to-face interactions, and with whom, more than simply physical proximity to others.
The audio sensors provide a powerful way to quantify verbal interactions. When matched with the proximity sensors, they can detect the amount of time that the wearer spends talking, and to whom, and the amount of time that someone else is talking to them. With that data, they can then quantify the duration and quality of social verbal interactions between specific individuals. These badges generally do not record the content of speech, i.e. what is actually said in the conversation, as that has more complex ethical and privacy implications. However, with current technology it would be possible to record speech content and transcribe into text output for analysis.
Overall, these badges can be used to quantify interpersonal interactions, of who is interacting with whom, how long they spend talking, and how long they spend listening. When paired with the teacher in a classroom, they can quantify the amount of time that the teacher spends in close proximity with each individual or group in the classroom, how long they spend talking nearby each group, and how long they spend listening nearby each group. They can even quantify how much time the students spend talking when the teacher is far away and when the teacher is in close proximity, and whether the teacher is engaged in those conversations.
Biometric Wristbands
Biometric wristbands provide measures of physical activity and physiological responses representing students’ biological states. They are usually lightweight bands worn on the wrist similar to a watch or fitness band. They will usually contain sensors to detect movement of the band, representing the student’s physical activity, and physiological sensors for detecting the student’s heart-rate and sometimes skin sweating response, or electrodermal activity, representing the student’s physiological arousal state.
A person’s physiological arousal level is measurable by physical effects in their body as they go from being disengaged, bored, or sleepy, to alert and motivated, and then to overly stressed and anxious. As physiological arousal level increases, when people become more cognitively engaged and then nervous, stressed or anxious, their heart rate increases and the skin on the palms of their hands and feet begins to sweat. This is caused by action of the autonomic nervous system that is driven by the brainstem in the lower parts of the brain.
Cognitive performance is related to physiological arousal level in a well-known U-shaped relationship called the Yerkes-Dodson Law. On this curve, cognitive performance is optimal at mid-levels of physiological arousal when people are alert, motivated, and engaged, rather than at the lower end when people are bored or sleepy and at the upper end when people are overly stressed and anxious.
By measuring changes in heart-rate and the skin sweating responses on the wrist, near the palm of the hand, these biometric wristbands can give a measure of the student’s changing physiological arousal level throughout learning activities in the classroom. One limitation of these devices is that they are best suited for measurement of dynamic changes in arousal level in response to particular events or interventions, rather than quantifying whether students are overall in a high or low state of arousal.
The devices are very sensitive to dynamic changes as students increase or decrease in arousal level in response to a particular event, but because of the U-shaped relationship between arousal level and cognitive performance, these increases and decreases can be difficult to interpret. For example, a relative increase in arousal level will be detrimental for students if they are already in a high state of arousal, thereby becoming more stressed and anxious, but will be beneficial to students who are in a relatively low state of arousal. These baseline levels of static arousal state are difficult to determine.
Nonetheless biometric wristbands can provide measures of relative changes in students’ physiological arousal levels, representing cognitive engagement or stress responses to events in the classroom. They can show whether physiological arousal levels increase or decrease with particular types of pedagogical practice or with particular student-student or student-teacher interactions. When paired with devices such as the sociometric badges, they can be used to assess how students’ arousal level changes when they are engaged in discussion or conversation, or when they are listening or quiet. They can also quantify changes in the student’s arousal level when they are in proximity with and interacting with specific students or groups, and when the teacher is close by and engaged in interaction or when the teacher is further away.
Wireless EEG brain measurement
These devices consist of headbands or headsets that contain sensors that connect with the skin on the head and detect changes in electrical activity of the brain directly. Although wireless versions of these headsets, and the intense computational analysis that is required, are the least developed for education research, they are amongst the most heavily marketed devices for home and classroom brain activity measurement. They are more obtrusive than wearing simple wristbands or badges, as they must be worn on the head and connect with the student’s scalp, but they can provide direct measurements of the brain states of the learners.
When neurons of the brain are active and “fire” their signal to other neurons, they generate very small electrical signals that can be detected by sensors on the skin of the head. This is known as the electroencephalogram or EEG. These EEG methods have been used in laboratory research for many decades to examine brain function during cognitive tasks and in various neurological and psychiatric conditions. EEG measurement is also a major tool in sleep research and clinical sleep assessment, as the different frequency bands of EEG brain activity change in characteristic ways over different levels from light to deep sleep.
Current research is also attempting to decode brain EEG signals to readout a person’s emotional state or level of cognitive engagement at any point in real-time. Those signals can then be displayed as feedback to the wearer so that they can adjust their behavior and learn to control their state (neurofeedback) or can be used to control external devices from brain activity directly (brain-computer interface)5.
A major limitation of these techniques in school classrooms is the compromise that is required in the hardware to be viable for everyday classroom studies as opposed to use in the neuroscience or clinical laboratory. The electrical activity signals detected from the brain are extremely weak and the electrode sensors used in EEG studies are extremely sensitive also to muscle activity of the forehead, eyes (particularly blinking), jaw, and neck, as well as picking up outside electrical interference from mains powered devices such as computers and monitors. In the neuroscience laboratory, all of these factors can be controlled so that interference with actual brain activity measurement is minimized, but this is far more challenging in a natural school classroom environment.
In EEG laboratories, electrode sensors are also typically “wet” systems using conductive gels to better connect between the sensors and the scalp and again to minimize “noise” or interference between the brain activity measurement and other sources of electrical signals. These typically take many minutes for an experienced technician to apply and set up. Manufacturers of wireless headset systems, however, are making huge engineering advances in “dry” electrode systems that can be easily and rapidly attached without the use of conductive gels, but this technology is still developing.
Once these wireless EEG headset systems are reliable for low-noise measurement in the difficult classroom environment, and viable for the wearer to rapidly attach to their head themselves, there is much exciting research that can progress for directly examining the brain states of learners during learning activities.
Computational Methods and Connectivity Analyses
A major challenge with all of these devices is with the computational methods required to answer research questions, or to provide simple metrics that are meaningful and useful in relation to classroom learning or pedagogical practice. Sociometric measures of inter-personal and verbal interactions, as well as physiological and brain states from biometric bands and EEG headsets during learning, have been correlated with other educational research measures. For example, we can examine relationships between these biometric measures and classroom observation studies of student behavior and engagement, or questionnaire and survey measures of students’ beliefs, motivations, and attitudes, as well as academic abilities or learning outcomes2.
These biometric measures can also be examined with “connectivity” analysis methods to examine the level of shared “synchrony” in physiological or brain states between students during classroom learning. These are multivariate computational methods that have been developed for social network analysis and for some forms of brain connectivity analysis, but can equally be applied to simultaneous measurement of biometric data from multiple students engaged in a classroom together.
With these methods, we examine periods of “synchrony” in physiological data or brain states, when multiple students are responding biologically in the same way at the same time to events in the classroom. When physiological or brain states are fluctuating in synchrony between students, so that they are sharing similar states, we would say that they are commonly engaged in the same events in the classroom. Similarly, if a student has biometric signals that are very different from others in the class, it is unlikely that they are engaged in the class in the same way as the other students. This is a powerful technique to quantify how “connected” students are with each other or with the ongoing events in the classroom.
The connectivity metrics can be quantified and examined either for individual students or for the group as a whole. For example, for individual students, we can examine how connected they are with other specific students or groups at any point in time. We can then examine relationships between their connectedness in physiological synchrony and their social interactions, overall arousal level, or academic abilities and learning outcomes. For the group as a whole, a group connectedness measure can tell us the extent to which all students are commonly engaged and driven by the same process, perhaps as with whole-class teacher-led instruction, and may show when students become disengaged or involved in their own individual thoughts or activities.
The development of these computational techniques is just as crucial as the hardware development of the various sensors reviewed above. Both aspects of hardware and computational analytic techniques must come together for progress in this area of wearable technology for neuroscience in classrooms.
Research Tools or Teacher Aids
At present, wearable technologies are at the forefront of research in educational neuroscience. Further developments will be required to improve their viability as research tools used to tell us about the social interactions, physiological responses, and neurologic brain states of learners during learning.
The hardware for sensors and the computational analytic techniques in this area are developing rapidly. One of the main challenges, however, is still to provide simple but powerful metrics that are useful and meaningful to educators in relation to student engagement or pedagogical practice.
Such measures will certainly come in the near future. That of course will open possibilities for wearable technologies to be used as additional aids to teachers or to schools to track various aspects of student engagement and performance during classroom instruction.
As these wearable technologies are being developed and will be increasingly marketed for use in schools, the challenge for educators is to consider how they can be adapted and used most effectively if they are to really benefit teaching and learning.
References
- Goh, F., Carroll, A. & Gillies, R. A review of the use of portable technologies as observational aids in the classroom. Information and Learning Sciences 120, 228-241 (2019).
- Gillies, R. et al. Multimodal representations during an inquiry problem-solving activity in a Year 6 science class: A case study investigating cooperation, physiological arousal and belief states. Australian Journal of Education 60, 111-127 (2016).
- Xu, J. & Zhong, B. Review on portable EEG technology in educational research. Computers in Human Behavior 81, 340-349 (2018).
- Wearable Tech – Thematic Research – GlobalData Report Store. Globaldata.com (2019). at <https://www.globaldata.com/store/report/gdtmt-tr-s219–wearable-tech-thematic-research/.>
- Gruzelier, J. EEG-neurofeedback for optimising performance. II: Creativity, the performing arts and ecological validity. Neuroscience & Biobehavioral Reviews 44, 142-158 (2013).